Open Access
PROCEEDINGS
Strengthening Mechanical Performance with Robust and Efficient Machine Learning-Assisted Path Planning for Additive Manufacturing of Continuous Fiber Composites
1 School of System Design and Intelligent Manufacturing, Southern University of Science and Technology, Shenzhen, 518055, China
* Corresponding Author: Yi Xiong. Email:
The International Conference on Computational & Experimental Engineering and Sciences 2024, 30(3), 1-1. https://doi.org/10.32604/icces.2024.011371
Abstract
Additive manufacturing of continuous fiber composites is an emerging field that enables the tunable mechanical performance of composite structure by flexibly controlling the spatial layout of continuous fibers. Transverse isotropic strengthening is advantageous property of continuous fiber, which is favorable to align with the principal stress orientation. However, the accuracy and efficiency of traditional methods for calculating principal stress field are unguaranteed due to the inherent complexity and variability of geometries, material properties, and operational conditions in additive manufacturing. Therefore, a machine learning-assisted path planning method is proposed to robustly and efficiently generate the continuous fiber path that significantly strengthen the mechanical performance of composite structure. The method employs a convolutional neural network enhanced with a self-attention mechanism to accurately predict principal stress fields from complex geometries, diverse material properties, and various operational conditions, ensuring robustness and efficiency of path generation. Subsequently, a wave projection function maps the infill morphology and ratio in alignment with the principal stress field distribution, enabling the generation of continuous fiber paths with minimized cutting points by solving the traveling salesman problem. Upon evaluating the method across a range of cantilever structures under diverse operational conditions and materials, the path generation efficiency remained consistent, and the structures fabricated exhibited superior mechanical performance compared to those with conventional Zig-Zag infill pattern. The method's robustness, efficiency, and effectiveness in strengthening mechanical performance render it highly suitable for real-world industrial applications.Keywords
Cite This Article
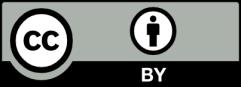
This work is licensed under a Creative Commons Attribution 4.0 International License , which permits unrestricted use, distribution, and reproduction in any medium, provided the original work is properly cited.