Open Access
PROCEEDINGS
A Digital Twin Framework for Structural Strength Monitoring
1 Dalian University of Technology, Dalian, 116024, China
* Corresponding Author: Kuo Tian. Email:
The International Conference on Computational & Experimental Engineering and Sciences 2024, 30(2), 1-1. https://doi.org/10.32604/icces.2024.011245
Abstract
Considering experimental testing data is costly, and sensor data is often sparse, while simulation analysis provides overall strength information with lower accuracy, a digital twin framework is proposed for full-field structural strength assessment and prediction. The framework is mainly divided into two stages. In the offline stage, the simulation model of the structure is established, and the sensor layouts are completed. Then, the DNN pre-training model is constructed based on the reduced simulation data. In the online stage, the experimentally measured data are predicted to obtain the time-series sensors data, and the traditional transfer learning multi-source data fusion model is constructed. Finally, to obtain the more accurate and robust digital twin model, the prediction error of the transfer learning model is fixed. To demonstrate the effectiveness of the proposed digital twin strength monitoring framework, two different engineering examples involving the plate with hole and the hierarchical stiffened plate are studied. The results indicate that the proposed method can establish an accurate digital twin model, achieve accurate interpolation of the sensor data, and provide a better prediction for the next loading step of the experiment. This provides a solution for achieving accurate real-time monitoring and predicting structural strength trends during the test.Keywords
Cite This Article
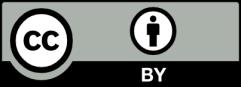
This work is licensed under a Creative Commons Attribution 4.0 International License , which permits unrestricted use, distribution, and reproduction in any medium, provided the original work is properly cited.