Open Access
PROCEEDINGS
High-Resolution Flow Field Reconstruction Based on Graph-Embedding Neural Network
1 Faculty of Environment and Life, Beijing University of Technology, Beijing, 100124, China
2 State Key Laboratory of Multiphase Flow in Power Engineering, Xi'an Jiaotong University, Xi'an, 710049, China
3 School of Chemical Engineering and Technology, Xi’an Jiaotong University, Xi’an, 710049, China
4 School of Mechanical Engineering, Beijing Institute of Petrochemical Technology, Beijing, 102617, China
* Corresponding Author: Weixin Jiang. Email:
The International Conference on Computational & Experimental Engineering and Sciences 2024, 30(1), 1-3. https://doi.org/10.32604/icces.2024.011266
Abstract
High resolution flow field results are of great significance for exploring physical laws and guiding practical engineering practice. However, traditional activities based on experiments or direct numerical solutions to obtain high-resolution flow fields typically require a significant amount of computational time or resources. In response to this challenge, this study proposes an efficient and robust high-resolution flow field reconstruction method by embedding graph theory into neural networks, to adapt to low data volume situations. In the high resolution flow field reconstruction problem of an NS equation, the proposed model has a lower mean squared error on the test set compared that of physics-informed neural network and classical neural network models. Among them, the low resolution data comes from 0.1% high resolution data. And the predicted results of the proposed model at time 10s is also closest to the direct numerical simulation results. The proposed model exhibits great advantages in robustness.Keywords
Cite This Article
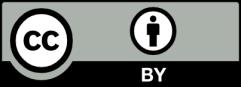
This work is licensed under a Creative Commons Attribution 4.0 International License , which permits unrestricted use, distribution, and reproduction in any medium, provided the original work is properly cited.