Open Access
PROCEEDINGS
Physics-Informed Neural Network for Young-Laplace Equation
1 International Research Center for Computational Mechanics, State Key Laboratory of Structural Analysis, Optimization and CAE Software for Industrial Equipment, Department of Engineering Mechanics, Faculty of Vehicle Engineering and Mechanics, Dalian University of Technology, Dalian, 116024, China
2 State Key Laboratory of Fine Chemicals, Frontier Science Center for Smart Materials Oriented Chemical Engineering, School of Chemistry, Dalian University of Technology, Dalian, 116024, China
3 DUT-BSU Joint Institute, Dalian University of Technology, Dalian, 116024, China
* Corresponding Author: Hongfei Ye. Email:
The International Conference on Computational & Experimental Engineering and Sciences 2024, 30(1), 1-1. https://doi.org/10.32604/icces.2024.011132
Abstract
Capillarity is prevalent in nature, daily life, and industrial processes, governed by the fundamental Young-Laplace equation. Solving this equation not only enhances our understanding of natural phenomena but also provides valuable insights into industrial advancements. To address challenges posed by conventional numerical methods in parameter identification and complex boundary condition handling, the Young-Laplace Physics-informed Neural Network (Y-L PINN) is introduced to solve the Young-Laplace equation within a tubular domain. Through computational analyses focusing on the classical capillary rise case, the proposed method's accuracy is affirmed through comparisons with Jurin's law, experimental data, and numerical results. The Y-L PINN method excels in parameter identification, e.g., contact angle, bond number and so on. Notably, numerical examples demonstrate its excellent predictive ability even in the presence of noisy data. For complex boundaries, the Y-L PINN method proves convenient in obtaining liquid meniscus shapes in vessels, aligning well with experimental results. Further exploration delves into the variation of the meniscus profile concerning wetting conditions or discontinuous boundaries. Importantly, the Y-L PINN method can directly solve the Young-Laplace equation with a discontinuous wetting boundary, eliminating the need for additional techniques. This work provides valuable insights for material wettability assessments, microstructure preparation, and advances in microfluidics research, contributing to the broader understanding and application of capillarity-related phenomena.Keywords
Cite This Article
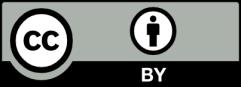
This work is licensed under a Creative Commons Attribution 4.0 International License , which permits unrestricted use, distribution, and reproduction in any medium, provided the original work is properly cited.