Open Access
PROCEEDINGS
Series-Parallel Machine Learning-Generated Five-Site Water Models for Ice Ih and Liquid: TIP5P-BG and TIP5P-BGT
1 International Research Center for Computational Mechanics, State Key Laboratory of Structural Analysis, Optimization and CAE Software for Industrial Equipment, Department of Engineering Mechanics, School of Mechanics and Aerospace Engineering, Dalian University of Technology, Dalian, 116024, China
* Corresponding Author: Jian Wang. Email:
The International Conference on Computational & Experimental Engineering and Sciences 2024, 29(3), 1-2. https://doi.org/10.32604/icces.2024.010916
Abstract
Icing is a ubiquitous phenomenon in nature and widely observed in the micro/nanoconfinement, e.g., two-dimensional ice growth on Au surface, nanoconfinement-induced phase change, nanodroplet freezing on surface, etc. These complicated and abstruse processes and behaviours demand deep understanding from the microscale level by the aid of molecular dynamics (MD) simulation [1]. However, it is still a great challenge to accurately describe the ice and liquid water simultaneously with the present water models [1,2]. In response to this, we propose a series-parallel machine learning (ML) approach consisting of classification back-propagation neural network (BPNN), parallel regression BPNNs and genetic algorithm to establish two most accurate models TIP5P-BG and TIP5P-BGT up to now. The established water models exhibit comprehensive balance among the crucial physical properties of ice and liquid water (melting point, density, vaporization enthalpy, self-diffusion coefficient and viscosity) with mean absolute percentage errors of 2.65% and 2.40%. The errors are significantly lower than the corresponding errors of 6.99% for TIP5P, 7.98% for TIP5P-E and 22.19% for TIP4P/Ice, which is commonly utilized for simulating ice. Moreover, the simulation results on the ice critical nucleus size and ice growth rate are excellently consistent with experiments. The present work also demonstrates the potential of the series-parallel ML method in the establishment of the intricate high-accuracy MD-based molecular model. More importantly, the most accurate models established in this work will be of interest to a broad readership in the fields for the molecular simulations of the phase transition and icing in nanoconfinement.Keywords
Cite This Article
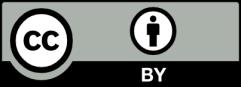
This work is licensed under a Creative Commons Attribution 4.0 International License , which permits unrestricted use, distribution, and reproduction in any medium, provided the original work is properly cited.