Open Access
PROCEEDINGS
Thermodynamically-Consistent NVT Flash Calculation and Thermodynamics-Informed Neural Network (TINN) Accelerating Phase Equilibrium Estimates
1 Computational Transport Phenomena Laboratory (CTPL), Division of Physical Sciences and Engineering (PSE), King
Abdullah University of Science and Technology (KAUST), Thuwal, 23955-6900, Saudi Arabia
2 PipeChina, Beijing, 100013, China
* Corresponding Author: Shuyu Sun. Email:
The International Conference on Computational & Experimental Engineering and Sciences 2023, 26(2), 1-2. https://doi.org/10.32604/icces.2023.09050
Abstract
The current well-developed and widely-recognized models describing interfacial tension are explicit functions of volume, temperature and moles so that it is more straightforward to compute the derivative of interfacial tension with respect to volume than pressure. We will propose a novel and comprehensive multicomponent multi-phase flow simulation algorithms based on diffuse interface models. In order to improve the robustness and reliability, realistic EoSs, represented by Peng-Robinson equation of states, are selected to formulate the thermodynamic correlations and an NVT-based flash calculation scheme is used to control the phase behaviors [1]. Compared to the minimized Gibbs free energy often used in NPT-based flash schemes, Helmholtz free energy will be minimized in our flash framework for further derivations. The ideal routine will start from the first law of thermodynamics to construct the entropy balance formulation, and the Helmholtz free energy density transport equation can be derived based on that. The energy dissipation in the whole system along with time needs to be proved to show the consistency between our model and general thermodynamic rules. For the numerical solutions to the model, we plan to construct an efficient convex-concave splitting approach targeting the Helmholtz free energy density, which is believed to efficiently handle the strong nonlinearity analyzed above, as well as the tight coupling between fluid velocity and molar densities. Generally, for a NVT type framework, the phase-wise mole compositions as well as volume are chosen as the primary variables arbitrarily, and we need to involve other aforementioned constraints resulted from thermodynamic rules and mass conservations to determine the counterparts in the other phase. Recently we demonstrated that the deep neural network models, while preserving high accuracy, are more than two hundred times faster than the conventional flash algorithms for multicomponent mixtures [2]. Previous machine learning methods assume a fixed number of components in the fluid mixture, which makes such models to have very limited practical usefulness. In this work, we propose to develop self-adaptive deep learning methods for general flash calculations, which can automatically determine the total number of phases existing in the multicomponent fluid mixture and related thermodynamic properties at equilibrium. Our preliminary work showed that, for example, the deep learning model with the 8-component Eagle Ford oil flash calculation results as training data accurately predicts the phase equilibrium properties of a 14-component Eagle Ford fluid mixture.Cite This Article
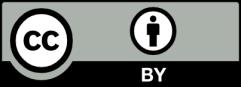
This work is licensed under a Creative Commons Attribution 4.0 International License , which permits unrestricted use, distribution, and reproduction in any medium, provided the original work is properly cited.