Open Access
ABSTRACT
Data Assimilation for Grain Growth Prediction via Multi-Phase-Field Models
* Corresponding Author: Hiromichi Nagao. Email: -tokyo.ac.jp.
The International Conference on Computational & Experimental Engineering and Sciences 2019, 22(2), 127-127. https://doi.org/10.32604/icces.2019.05384
Abstract
Data assimilation (DA) is a computational technique to integrate numerical simulation models and observational/experimental data based on Bayesian statistics. DA is accepted as an essential methodology for the modern weather forecasting, and is applied to various fields of science including structural materials science. We propose a DA methodology to evaluate unobservable parameters involved in multi-phase-field models with the aim of accurately predicting the observed grain growth, such as in metals and alloys. This approach integrates models and a set of observational image data of grain structures. Since the set of image data is not a time series, directly applying conventional DA techniques that require time series as the input data is difficult. Our key idea is to construct a time series with an appropriate statistic that characterizes static image data of grain structures. The empirical Bayes method estimates not only a probability density function of the parameters but also an initial phase-field, which is generally unobservable in real experiments. The proposed method is confirmed to estimate, from real experimental images of grain structures in a steel alloy, unobservable parameters together with their uncertainties, and successfully selects the initial phase-field that best explains the experimental data from among candidate initial phase-fields.Cite This Article
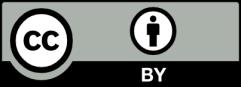
This work is licensed under a Creative Commons Attribution 4.0 International License , which permits unrestricted use, distribution, and reproduction in any medium, provided the original work is properly cited.