Open Access
ABSTRACT
Image Processing/Machine-Learning for Auto-Labeling of Steel Images on Present Microstructures
Research and Services Division of Materials Data and Integrated System, National Institute for Materials Science, Tsukuba, Japan.
School of Engineering, University of Tokyo, Tokyo, Japan.
Research Center for Advanced Science and Technology, University of Tokyo, Tokyo, Japan.
*Corresponding Author: Dmitry S. Bulgarevich. Email: .
The International Conference on Computational & Experimental Engineering and Sciences 2019, 22(2), 122-122. https://doi.org/10.32604/icces.2019.05271
Abstract
The microstructure of steel greatly determines its mechanical properties/performance and holds information on chemical composition and processing history. Therefore, quantitative analysis of optical or SEM images on formed microstructure phases is one of the primary interests for metallurgy. So far, such analyses in laboratories are done manually by experts and are very time consuming. However, with modern microscopy techniques of automated image acquisitions over the large imaging areas and even by using of sample slicing for three-dimensional imaging, the amount of image data could be overwhelming for manual examinations. In this respect, there is a possibility that machine learning (ML) could help to automate such routine tasks and even unveil the hidden materials paradigms. In this study, we demonstrate that optical microscopy images of steel materials could be effectively labeled on ferrite/perlite, ferrite/perlite/bainite, and bainite/martensite type microstructures with image pre-processing and statistical analysis including several ML techniques. Though several popular ML classifiers were able to get reasonable labeling accuracy, the random forest one was the best choice in terms of overall performance/robustness/usability. This image labeling with well-trained classifier together with our recently reported classifiers for image segmentations can be used even by non-expert in microstructural phase analysis for accurate estimation of microstructure types and corresponding phase contents in steels. This work was supported by Council for Science, Technology and Innovation (CSTI), Cross-ministerial Strategic Innovation Promotion Program (SIP), “Structural Materials for Innovation” and “Materials Integration for Revolutionary Design System of Structural Materials” (Funding agency: JST).Cite This Article
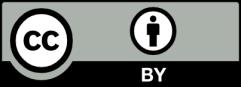
This work is licensed under a Creative Commons Attribution 4.0 International License , which permits unrestricted use, distribution, and reproduction in any medium, provided the original work is properly cited.