Open Access
ABSTRACT
Automated Segmentation of Atherosclerotic Plaque Using Bayes Classifier for Multi-Contrast In Vivo and Ex Vivo MR Images
Mathematics Department, Worcester Polytechnic Institute, Worcester, MA 01609, USA
Mathematics Department, Beijing Normal University, Beijing, China
Mallinkcrodt Institute of Radiology, Washington University, St. Louis, MO 63110, USA
The International Conference on Computational & Experimental Engineering and Sciences 2007, 1(1), 29-34. https://doi.org/10.3970/icces.2007.001.029
Abstract
Atherosclerotic plaques may rupture without warning and cause acute cardiovascular syndromes such as heart attack and stroke. Accurate identification of plaque components will improve the accuracy and reliability of computational models. In this article, we present a segmentation method using a cluster analysis technique to quantify and classify plaque components from magnetic resonance images (MRI). 3D in vivo and ex vivo multi-contrast (T1-, proton density-, and T2-weighted) MR Images were acquired from a patient of cardiovascular disease. Normal distribution Bayes classifier was performed on ex vivo and in vivo MR Images respectively. The resulting segmentation obtained from cluster analysis showed very good agreement with histological data. 3D visualization of the plaque was presented. Combination of in vivo and ex vivo MRI data enabled us to quantify the shrinkage between the ex vivo plaque sample and its in vivo state. For this patient, the average shrinkage is 9.14% at cross section and 33.33% in axial direction. This information is essential to determining proper initial stress/strain conditions for computational plaque models.Cite This Article
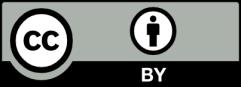
This work is licensed under a Creative Commons Attribution 4.0 International License , which permits unrestricted use, distribution, and reproduction in any medium, provided the original work is properly cited.