Open Access
ARTICLE
Deep Learning Empowered Diagnosis of Diabetic Retinopathy
1 Department of Computer Engineering, College of Computer Science and Information Technology, Imam Abdulrahman Bin Faisal University, P.O. Box 1982, Dammam, 31441, Saudi Arabia
2 Department of Computer Science, College of Computer Science and Information Technology, Imam Abdulrahman Bin Faisal University, P.O. Box 1982, Dammam, 31441, Saudi Arabia
* Corresponding Author: Atta Rahman. Email:
Intelligent Automation & Soft Computing 2025, 40, 125-143. https://doi.org/10.32604/iasc.2025.058509
Received 14 September 2024; Accepted 20 December 2024; Issue published 23 January 2025
Abstract
Diabetic retinopathy (DR) is a complication of diabetes that can lead to reduced vision or even blindness if left untreated. Therefore, early and accurate detection of this disease is crucial for diabetic patients to prevent vision loss. This study aims to develop a deep-learning approach for the early and precise diagnosis of DR, as manual detection can be time-consuming, costly, and prone to human error. The classification task is divided into two groups for binary classification: patients with DR (diagnoses 1–4) and those without DR (diagnosis 0). For multi-class classification, the categories are no DR, mild DR, moderate DR, severe DR, and proliferative diabetic retinopathy (PDR). To achieve this, the proposed model utilizes two pre-trained convolutional neural networks (CNNs), specifically ResNet50 and DenseNet-121. Both models were trained and evaluated on fundus images sourced from the widely recognized APTOS dataset, a publicly available resource., and achieved impressive training and testing accuracies. For binary classification, DenseNet-121 achieved an accuracy of 98.1%, while ResNet50 attained an accuracy of 97.4%. In multi-class classification for DR, DenseNet-121 achieved an accuracy of 82.0%, and ResNet50 reached an accuracy of 80.8%. The results are promising and comparable to state-of-the-art techniques in the literature for both binary and multi-label classification of DR.Keywords
Cite This Article
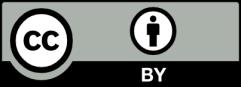
This work is licensed under a Creative Commons Attribution 4.0 International License , which permits unrestricted use, distribution, and reproduction in any medium, provided the original work is properly cited.