Open Access
ARTICLE
Enhancing Network Security: Leveraging Machine Learning for Integrated Protection and Intrusion Detection
1 Ministry of Higher Education and Scientific Research, Baghdad, 10011, Iraq
2 College of Nursing, Department of Basic Nursing Sciences, University of Kirkuk, Kirkuk, 36001, Iraq
3 Computer Science Department, Bayan University, Erbil, 44001, Kurdistan, Iraq
4 Artificial Intelligence Engineering Department, College of Engineering, Al-Ayen University, Thi-Qar, 64001, Iraq
5 Symbiosis Institute of Technology (SIT) Pune Campus, Symbiosis International (Deemed University) (SIU), Pune, 412115, Maharashtra, India
* Corresponding Author: Saadaldeen Rashid Ahmed. Email:
Intelligent Automation & Soft Computing 2025, 40, 1-27. https://doi.org/10.32604/iasc.2024.058624
Received 17 September 2024; Accepted 20 November 2024; Issue published 10 January 2025
Abstract
This study introduces an innovative hybrid approach that integrates deep learning with blockchain technology to improve cybersecurity, focusing on network intrusion detection systems (NIDS). The main goal is to overcome the shortcomings of conventional intrusion detection techniques by developing a more flexible and robust security architecture. We use seven unique machine learning models to improve detection skills, emphasizing data quality, traceability, and transparency, facilitated by a blockchain layer that safeguards against data modification and ensures auditability. Our technique employs the Synthetic Minority Oversampling Technique (SMOTE) to equilibrate the dataset, therefore mitigating prevalent class imbalance difficulties in intrusion detection. The model selection procedure determined that Random Forest was the most successful model, with a notable detection accuracy of 97%. This substantially surpasses conventional methods and enhances the system’s capacity to identify both established and novel threats with exceptional accuracy. To optimize feature selection and maximize performance, we use Extreme Gradient Boosting (XGBoost), which improves the significance of chosen features while reducing the danger of overfitting. Our study indicates that the integrated use of machine learning for pattern identification, multi-factor authentication (MFA) for access security, and blockchain for data validation constitutes a thorough and sustainable cybersecurity solution. This architecture not only increases security but also lowers the need for regular human monitoring, significantly cutting energy consumption connected with cybersecurity infrastructure. The research finds that this integrated strategy provides a realistic road for increasing network security, addressing real-world cyber threats, and promoting eco-friendly practices in IT security.Keywords
Cite This Article
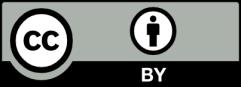
This work is licensed under a Creative Commons Attribution 4.0 International License , which permits unrestricted use, distribution, and reproduction in any medium, provided the original work is properly cited.