Open Access
ARTICLE
Overfitting in Machine Learning: A Comparative Analysis of Decision Trees and Random Forests
Department of Mathematics, Faculty of Mathematical and Natural Sciences, University of Prishtina, Prishtina, 10000, Kosovo
* Corresponding Author: Eliot Bytyçi. Email:
Intelligent Automation & Soft Computing 2024, 39(6), 987-1006. https://doi.org/10.32604/iasc.2024.059429
Received 08 October 2024; Accepted 10 December 2024; Issue published 30 December 2024
Abstract
Machine learning has emerged as a pivotal tool in deciphering and managing this excess of information in an era of abundant data. This paper presents a comprehensive analysis of machine learning algorithms, focusing on the structure and efficacy of random forests in mitigating overfitting—a prevalent issue in decision tree models. It also introduces a novel approach to enhancing decision tree performance through an optimized pruning method called Adaptive Cross-Validated Alpha CCP (ACV-CCP). This method refines traditional cost complexity pruning by streamlining the selection of the alpha parameter, leveraging cross-validation within the pruning process to achieve a reliable, computationally efficient alpha selection that generalizes well to unseen data. By enhancing computational efficiency and balancing model complexity, ACV-CCP allows decision trees to maintain predictive accuracy while minimizing overfitting, effectively narrowing the performance gap between decision trees and random forests. Our findings illustrate how ACV-CCP contributes to the robustness and applicability of decision trees, providing a valuable perspective on achieving computationally efficient and generalized machine learning models.Keywords
Cite This Article
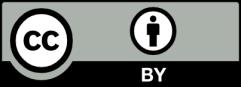
This work is licensed under a Creative Commons Attribution 4.0 International License , which permits unrestricted use, distribution, and reproduction in any medium, provided the original work is properly cited.