Open Access
ARTICLE
Evaluating the Effectiveness of Graph Convolutional Network for Detection of Healthcare Polypharmacy Side Effects
1 Collage of Engineering, Department of Electrical and Computer Engineering, Altinbas University, Istanbul, 34000, Turkey
2 College of Computer Science and Information Technology, Department of Information Technology, University of Kirkuk, Kirkuk, 36001, Iraq
* Corresponding Author: Omer Nabeel Dara. Email:
(This article belongs to the Special Issue: Medical Imaging Decision Support Systems Using Deep Learning and Machine Learning Algorithms)
Intelligent Automation & Soft Computing 2024, 39(6), 1007-1033. https://doi.org/10.32604/iasc.2024.058736
Received 19 September 2024; Accepted 20 November 2024; Issue published 30 December 2024
Abstract
Healthcare polypharmacy is routinely used to treat numerous conditions; however, it often leads to unanticipated bad consequences owing to complicated medication interactions. This paper provides a graph convolutional network (GCN)-based model for identifying adverse effects in polypharmacy by integrating pharmaceutical data from electronic health records (EHR). The GCN framework analyzes the complicated links between drugs to forecast the possibility of harmful drug interactions. Experimental assessments reveal that the proposed GCN model surpasses existing machine learning approaches, reaching an accuracy (ACC) of 91%, an area under the receiver operating characteristic curve (AUC) of 0.88, and an F1-score of 0.83. Furthermore, the overall accuracy of the model achieved 98.47%. These findings imply that the GCN model is helpful for monitoring individuals receiving polypharmacy. Future research should concentrate on improving the model and extending datasets for therapeutic applications.Keywords
Cite This Article
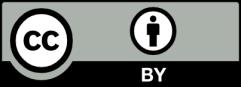
This work is licensed under a Creative Commons Attribution 4.0 International License , which permits unrestricted use, distribution, and reproduction in any medium, provided the original work is properly cited.