Open Access
ARTICLE
Enhanced Diagnostic Precision: Deep Learning for Tumors Lesion Classification in Dermatology
1 Engineering Technical College, Al-Ayen University, Thi-Qar, 64001, Iraq
2 Information Technology College, University of Babylon, Hilla, 51001, Iraq
3 Information and Communication Technology Research Group, Scientific Research Center, Al-Ayen University, Thi-Qar, 64001, Iraq
4 College of Computer Sciences and Information Technology, University of Kerbala, Karbala, 56001, Iraq
5 Artificial Intelligence Engineering Department, College of Engineering, Al-Ayen University, Thi-Qar, 64001, Iraq
6 Computer Science, Bayan University, Erbil, 44001, Iraq
* Corresponding Authors: Rafid Sagban. Email: ; Saadaldeen Rashid Ahmed. Email:
Intelligent Automation & Soft Computing 2024, 39(6), 1035-1051. https://doi.org/10.32604/iasc.2024.058416
Received 11 September 2024; Accepted 10 December 2024; Issue published 30 December 2024
Abstract
Skin cancer is a highly frequent kind of cancer. Early identification of a phenomenon significantly improves outcomes and mitigates the risk of fatalities. Melanoma, basal, and squamous cell carcinomas are well-recognized cutaneous malignancies. Malignant We can differentiate Melanoma from non-pigmented carcinomas like basal and squamous cell carcinoma. The research on developing automated skin cancer detection systems has primarily focused on pigmented malignant type melanoma. The limited availability of datasets with a wide range of lesion categories has hindered in-depth exploration of non-pigmented malignant skin lesions. The present study investigates the feasibility of automated methods for detecting pigmented skin lesions with potential malignancy. To diagnose skin lesions, medical professionals employ a two-step approach. Before detecting malignant types with other deep learning (DL) models, a preliminary step involves using a DL model to identify the skin lesions as either pigmented or non-pigmented. The performance assessments accurately assessed four distinct DL models: Long short-term memory (LSTM), Visual Geometry Group (VGG19), Residual Blocks (ResNet50), and AlexNet. The LSTM model exhibited higher classification accuracy compared to the other models used. The accuracy of LSTM for pigmented and non-pigmented, pigmented tumours and benign classes, and melanomas and pigmented nevus classes was 0.9491, 0.9531, and 0.949, respectively. Automated computerized skin cancer detection promises to enhance diagnostic efficiency and precision significantly.Keywords
Cite This Article
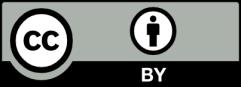
This work is licensed under a Creative Commons Attribution 4.0 International License , which permits unrestricted use, distribution, and reproduction in any medium, provided the original work is properly cited.