Open Access
ARTICLE
Predicting Grain Orientations of 316 Stainless Steel Using Convolutional Neural Networks
Mechanical Engineering Department, College of Engineering and Architecture, Umm Al-Qura University, Makkah, 21955, Saudi Arabia
* Corresponding Author: Ahmed R. Abdo. Email:
Intelligent Automation & Soft Computing 2024, 39(5), 929-947. https://doi.org/10.32604/iasc.2024.056341
Received 20 July 2024; Accepted 13 September 2024; Issue published 31 October 2024
Abstract
This paper presents a deep learning Convolutional Neural Network (CNN) for predicting grain orientations from electron backscatter diffraction (EBSD) patterns. The proposed model consists of multiple neural network layers and has been trained on a dataset of EBSD patterns obtained from stainless steel 316 (SS316). Grain orientation changes when considering the effects of temperature and strain rate on material deformation. The deep learning CNN predicts material orientation using the EBSD method to address this challenge. The accuracy of this approach is evaluated by comparing the predicted crystal orientation with the actual orientation under different conditions, using the Root-Mean-Square Error (RMSE) as the measure. Results show that changing the temperature causes different grain orientations to form, meeting the requirements. Further investigations were conducted to validate the results.Keywords
Cite This Article
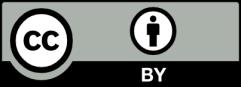
This work is licensed under a Creative Commons Attribution 4.0 International License , which permits unrestricted use, distribution, and reproduction in any medium, provided the original work is properly cited.