Open Access
ARTICLE
Robot Vision over CosGANs to Enhance Performance with Source-Free Domain Adaptation Using Advanced Loss Function
1 Department of Software Engineering, NED University of Engineering & Technology, Karachi, 75270, Pakistan
2 Department of Computer Science & Information Technology, NED University of Engineering & Technology, Karachi, 75270, Pakistan
3 Department of Computer Science, IQRA University, Karachi, 75500, Pakistan
* Corresponding Author: Rohail Qamar. Email:
Intelligent Automation & Soft Computing 2024, 39(5), 855-887. https://doi.org/10.32604/iasc.2024.055074
Received 15 June 2024; Accepted 05 August 2024; Issue published 31 October 2024
Abstract
Domain shift is when the data used in training does not match the ones it will be applied to later on under similar conditions. Domain shift will reduce accuracy in results. To prevent this, domain adaptation is done, which adapts the pre-trained model to the target domain. In real scenarios, the availability of labels for target data is rare thus resulting in unsupervised domain adaptation. Herein, we propose an innovative approach where source-free domain adaptation models and Generative Adversarial Networks (GANs) are integrated to improve the performance of computer vision or robotic vision-based systems in our study. Cosine Generative Adversarial Network (CosGAN) is developed as a GAN that uses cosine embedding loss to handle issues associated with unsupervised source-relax domain adaptations. For less complex architecture, the CosGAN training process has two steps that produce results almost comparable to other state-of-the-art techniques. The efficiency of CosGAN was compared by conducting experiments using benchmarked datasets. The approach was evaluated on different datasets and experimental results show superiority over existing state-of-the-art methods in terms of accuracy as well as generalization ability. This technique has numerous applications including wheeled robots, autonomous vehicles, warehouse automation, and all image-processing-based automation tasks so it can reshape the field of robotic vision with its ability to make robots adapt to new tasks and environments efficiently without requiring additional labeled data. It lays the groundwork for future expansions in robotic vision and applications. Although GAN provides a variety of outstanding features, it also increases the risk of instability and over-fitting of the training data thus making the data difficult to converge.Keywords
Cite This Article
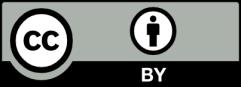
This work is licensed under a Creative Commons Attribution 4.0 International License , which permits unrestricted use, distribution, and reproduction in any medium, provided the original work is properly cited.