Open Access
ARTICLE
A Deep Transfer Learning Approach for Addressing Yaw Pose Variation to Improve Face Recognition Performance
1 Department of Electronics and Instrumentation Engineering, College of Engineering and Technology, SRM Institute of Science and Technology, Kattankulathur, Chennai, Tamil Nadu, 603203, India
2 Department of Electronics and Communication Engineering, SRM University, Amaravati, Mangalagiri, Andhra Pradesh, 522502, India
3 Security Electronics and Cyber Technology, Bhabha Atomic Research Centre, Anushakti Nagar, Mumbai, Maharashtra, 400085, India
* Corresponding Author: K. A. Sunitha. Email:
Intelligent Automation & Soft Computing 2024, 39(4), 745-764. https://doi.org/10.32604/iasc.2024.052983
Received 21 April 2024; Accepted 13 June 2024; Issue published 06 September 2024
Abstract
Identifying faces in non-frontal poses presents a significant challenge for face recognition (FR) systems. In this study, we delved into the impact of yaw pose variations on these systems and devised a robust method for detecting faces across a wide range of angles from 0° to ±90°. We initially selected the most suitable feature vector size by integrating the Dlib, FaceNet (Inception-v2), and “Support Vector Machines (SVM)” + “K-nearest neighbors (KNN)” algorithms. To train and evaluate this feature vector, we used two datasets: the “Labeled Faces in the Wild (LFW)” benchmark data and the “Robust Shape-Based FR System (RSBFRS)” real-time data, which contained face images with varying yaw poses. After selecting the best feature vector, we developed a real-time FR system to handle yaw poses. The proposed FaceNet architecture achieved recognition accuracies of 99.7% and 99.8% for the LFW and RSBFRS datasets, respectively, with 128 feature vector dimensions and minimum Euclidean distance thresholds of 0.06 and 0.12. The FaceNet + SVM and FaceNet + KNN classifiers achieved classification accuracies of 99.26% and 99.44%, respectively. The 128-dimensional embedding vector showed the highest recognition rate among all dimensions. These results demonstrate the effectiveness of our proposed approach in enhancing FR accuracy, particularly in real-world scenarios with varying yaw poses.Keywords
Cite This Article
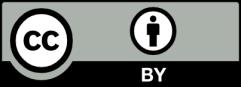
This work is licensed under a Creative Commons Attribution 4.0 International License , which permits unrestricted use, distribution, and reproduction in any medium, provided the original work is properly cited.