Open Access
ARTICLE
Improving Low-Resource Machine Translation Using Reinforcement Learning from Human Feedback
School of Information Science and Engineering, Yunnan University, Kunming, 650500, China
* Corresponding Author: Liqing Wang. Email:
Intelligent Automation & Soft Computing 2024, 39(4), 619-631. https://doi.org/10.32604/iasc.2024.052971
Received 20 April 2024; Accepted 08 July 2024; Issue published 06 September 2024
Abstract
Neural Machine Translation is one of the key research directions in Natural Language Processing. However, limited by the scale and quality of parallel corpus, the translation quality of low-resource Neural Machine Translation has always been unsatisfactory. When Reinforcement Learning from Human Feedback (RLHF) is applied to low-resource machine translation, commonly encountered issues of substandard preference data quality and the higher cost associated with manual feedback data. Therefore, a more cost-effective method for obtaining feedback data is proposed. At first, optimizing the quality of preference data through the prompt engineering of the Large Language Model (LLM), then combining human feedback to complete the evaluation. In this way, the reward model could acquire more semantic information and human preferences during the training phase, thereby enhancing feedback efficiency and the result’s quality. Experimental results demonstrate that compared with the traditional RLHF method, our method has been proven effective on multiple datasets and exhibits a notable improvement of 1.07 in BLUE. Meanwhile, it is also more favorably received in the assessments conducted by human evaluators and GPT-4o.Keywords
Cite This Article
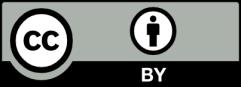
This work is licensed under a Creative Commons Attribution 4.0 International License , which permits unrestricted use, distribution, and reproduction in any medium, provided the original work is properly cited.