Open Access
ARTICLE
Mathematical Named Entity Recognition Based on Adversarial Training and Self-Attention
1 Key Laboratory of the Evaluation and Monitoring of Southwest Land Resources (Sichuan Normal University), Ministry of Education, Chengdu, 610166, China
2 School of Mathematics and Science, Sichuan Normal University, Chengdu, 610166, China
3 Chengdu State-Owned Jinjiang Machine Factory, Chengdu, 610100, China
* Corresponding Authors: Chun Yang. Email: ; Delin Zhang. Email:
Intelligent Automation & Soft Computing 2024, 39(4), 649-664. https://doi.org/10.32604/iasc.2024.051724
Received 13 March 2024; Accepted 28 June 2024; Issue published 06 September 2024
Abstract
Mathematical named entity recognition (MNER) is one of the fundamental tasks in the analysis of mathematical texts. To solve the existing problems of the current neural network that has local instability, fuzzy entity boundary, and long-distance dependence between entities in Chinese mathematical entity recognition task, we propose a series of optimization processing methods and constructed an Adversarial Training and Bidirectional long short-term memory-Selfattention Conditional random field (AT-BSAC) model. In our model, the mathematical text was vectorized by the word embedding technique, and small perturbations were added to the word vector to generate adversarial samples, while local features were extracted by Bi-directional Long Short-Term Memory (BiLSTM). The self-attentive mechanism was incorporated to extract more dependent features between entities. The experimental results demonstrated that the AT-BSAC model achieved a precision (P) of 93.88%, a recall (R) of 93.84%, and an F1-score of 93.74%, respectively, which is 8.73% higher than the F1-score of the previous Bi-directional Long Short-Term Memory Conditional Random Field (BiLSTM-CRF) model. The effectiveness of the proposed model in mathematical named entity recognition.Keywords
Cite This Article
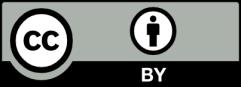
This work is licensed under a Creative Commons Attribution 4.0 International License , which permits unrestricted use, distribution, and reproduction in any medium, provided the original work is properly cited.