Open Access
ARTICLE
A Hierarchical Two-Level Feature Fusion Approach for SMS Spam Filtering
1 Computerized Intelligence Systems Laboratory, Department of Computer Engineering, University of Tabriz, Tabriz, 51368, Iran
2 Ministry of Education Iraq, General Direction of Vocational Education, Al-Najaf, 54001, Iraq
3 Department of Computer Engineering, University of Tabriz, Tabriz, 51368, Iran
* Corresponding Author: Mohammad-Reza Feizi-Derakhshi. Email:
Intelligent Automation & Soft Computing 2024, 39(4), 665-682. https://doi.org/10.32604/iasc.2024.050452
Received 07 February 2024; Accepted 27 May 2024; Issue published 06 September 2024
Abstract
SMS spam poses a significant challenge to maintaining user privacy and security. Recently, spammers have employed fraudulent writing styles to bypass spam detection systems. This paper introduces a novel two-level detection system that utilizes deep learning techniques for effective spam identification to address the challenge of sophisticated SMS spam. The system comprises five steps, beginning with the preprocessing of SMS data. RoBERTa word embedding is then applied to convert text into a numerical format for deep learning analysis. Feature extraction is performed using a Convolutional Neural Network (CNN) for word-level analysis and a Bidirectional Long Short-Term Memory (BiLSTM) for sentence-level analysis. The two-level feature extraction enables a complete understanding of individual words and sentence structure. The novel part of the proposed approach is the Hierarchical Attention Network (HAN), which fuses and selects features at two levels through an attention mechanism. The HAN can deal with words and sentences to focus on the most pertinent aspects of messages for spam detection. This network is productive in capturing meaningful features, considering both word-level and sentence-level semantics. In the classification step, the model classifies the messages into spam and ham. This hybrid deep learning method improve the feature representation, and enhancing the model’s spam detection capabilities. By significantly reducing the incidence of SMS spam, our model contributes to a safer mobile communication environment, protecting users against potential phishing attacks and scams, and aiding in compliance with privacy and security regulations. This model’s performance was evaluated using the SMS Spam Collection Dataset from the UCI Machine Learning Repository. Cross-validation is employed to consider the dataset’s imbalanced nature, ensuring a reliable evaluation. The proposed model achieved a good accuracy of 99.48%, underscoring its efficiency in identifying SMS spam.Keywords
Cite This Article
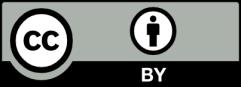
This work is licensed under a Creative Commons Attribution 4.0 International License , which permits unrestricted use, distribution, and reproduction in any medium, provided the original work is properly cited.