Open Access
ARTICLE
Importance-Weighted Transfer Learning for Fault Classification under Covariate Shift
1 Institute of Cyber-Systems and Control, Zhejiang University, Hangzhou, 310027, China
2 State Key Laboratory of Industrial Control Technology, Institute of Cyber-Systems and Control, Zhejiang University, Hangzhou, 310027, China
* Corresponding Author: Lei Xie. Email:
Intelligent Automation & Soft Computing 2024, 39(4), 683-696. https://doi.org/10.32604/iasc.2023.038543
Received 17 December 2022; Accepted 24 February 2023; Issue published 06 September 2024
Abstract
In the process of fault detection and classification, the operation mode usually drifts over time, which brings great challenges to the algorithms. Because traditional machine learning based fault classification cannot dynamically update the trained model according to the probability distribution of the testing dataset, the accuracy of these traditional methods usually drops significantly in the case of covariate shift. In this paper, an importance-weighted transfer learning method is proposed for fault classification in the nonlinear multi-mode industrial process. It effectively alters the drift between the training and testing dataset. Firstly, the mutual information method is utilized to perform feature selection on the original data, and a number of characteristic parameters associated with fault classification are selected according to their mutual information. Then, the importance-weighted least-squares probabilistic classifier (IWLSPC) is utilized for binary fault detection and multi-fault classification in covariate shift. Finally, the Tennessee Eastman (TE) benchmark is carried out to confirm the effectiveness of the proposed method. The experimental result shows that the covariate shift adaptation based on importance-weight sampling is superior to the traditional machine learning fault classification algorithms. Moreover, IWLSPC can not only be used for binary fault classification, but also can be applied to the multi-classification target in the process of fault diagnosis.Keywords
Cite This Article
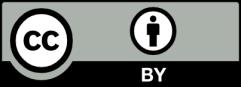
This work is licensed under a Creative Commons Attribution 4.0 International License , which permits unrestricted use, distribution, and reproduction in any medium, provided the original work is properly cited.