Open Access
ARTICLE
Multi-Layer Feature Extraction with Deformable Convolution for Fabric Defect Detection
1 School of Computer Science, Nanjing University of Information Science and Technology, Nanjing, 210044, China
2 State Key Lab. for Novel Software Technology, Nanjing University, Nanjing, 210023, China
3 Engineering Research Center of Digital Forensics, Ministry of Education, Nanjing University of Information Science and Technology, Nanjing, 210044, China
4 Jiangsu Collaborative Innovation Center of Atmospheric Environment and Equipment Technology (CICAEET), Nanjing University of Information Science and Technology, Nanjing, 210044, China
5 College of Mathematics and Information Science, Nanjing Normal University of Special Education, Nanjing, 210038, China
* Corresponding Author: Jielin Jiang. Email:
Intelligent Automation & Soft Computing 2024, 39(4), 725-744. https://doi.org/10.32604/iasc.2024.036897
Received 15 October 2022; Accepted 06 December 2022; Issue published 06 September 2024
Abstract
In the textile industry, the presence of defects on the surface of fabric is an essential factor in determining fabric quality. Therefore, identifying fabric defects forms a crucial part of the fabric production process. Traditional fabric defect detection algorithms can only detect specific materials and specific fabric defect types; in addition, their detection efficiency is low, and their detection results are relatively poor. Deep learning-based methods have many advantages in the field of fabric defect detection, however, such methods are less effective in identifying multi-scale fabric defects and defects with complex shapes. Therefore, we propose an effective algorithm, namely multi-layer feature extraction combined with deformable convolution (MFDC), for fabric defect detection. In MFDC, multi-layer feature extraction is used to fuse the underlying location features with high-level classification features through a horizontally connected top-down architecture to improve the detection of multi-scale fabric defects. On this basis, a deformable convolution is added to solve the problem of the algorithm’s weak detection ability of irregularly shaped fabric defects. In this approach, Roi Align and Cascade-RCNN are integrated to enhance the adaptability of the algorithm in materials with complex patterned backgrounds. The experimental results show that the MFDC algorithm can achieve good detection results for both multi-scale fabric defects and defects with complex shapes, at the expense of a small increase in detection time.Keywords
Cite This Article
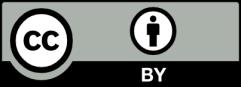
This work is licensed under a Creative Commons Attribution 4.0 International License , which permits unrestricted use, distribution, and reproduction in any medium, provided the original work is properly cited.