Open Access
ARTICLE
Ensemble Modeling for the Classification of Birth Data
1 Department of Information Technology, University of Gujrat, Gujrat, 50700, Pakistan
2 School of Digital Convergence Business, University of Central Punjab, Rawalpindi, 46000, Pakistan
3 Faculty of Computing, The Islamia University of Bahawalpur, Bahawalpur, 63100, Pakistan
4 College of Computer Science and Information Technology, Al Baha University, Al Baha, 1988, Saudi Arabia
5 School of Computer Science and Engineering, Yeungnam University, Gyeongsan, 38541, Republic of Korea
6 Faculty of Engineering, Uni de Moncton, Moncton, NB E1A3E9, Canada
7 International Institute of Technology and Management, Commune d’Akanda, Libreville, 1989, Gabon
8 Department of Electrical and Electronic Engineering Science, School of Electrical Engineering, University of Johannesburg, Johannesburg, 2006, South Africa
9 Spectrum of Knowledge Production & Skills Development, Sfax, 3027, Tunisia
10 College of Computer Science and Engineering, University of Ha’il, Ha’il, 55476, Saudi Arabia
11 College of Science, Zagazig University, Zagazig, 44511, Egypt
* Corresponding Author: Muhammad Shafiq. Email:
Intelligent Automation & Soft Computing 2024, 39(4), 765-781. https://doi.org/10.32604/iasc.2023.034029
Received 04 July 2022; Accepted 19 May 2023; Issue published 06 September 2024
Abstract
Machine learning (ML) and data mining are used in various fields such as data analysis, prediction, image processing and especially in healthcare. Researchers in the past decade have focused on applying ML and data mining to generate conclusions from historical data in order to improve healthcare systems by making predictions about the results. Using ML algorithms, researchers have developed applications for decision support, analyzed clinical aspects, extracted informative information from historical data, predicted the outcomes and categorized diseases which help physicians make better decisions. It is observed that there is a huge difference between women depending on the region and their social lives. Due to these differences, scholars have been encouraged to conduct studies at a local level in order to better understand those factors that affect maternal health and the expected child. In this study, the ensemble modeling technique is applied to classify birth outcomes based on either cesarean section (C-Section) or normal delivery. A voting ensemble model for the classification of a birth dataset was made by using a Random Forest (RF), Gradient Boosting Classifier, Extra Trees Classifier and Bagging Classifier as base learners. It is observed that the voting ensemble modal of proposed classifiers provides the best accuracy, i.e., 94.78%, as compared to the individual classifiers. ML algorithms are more accurate due to ensemble models, which reduce variance and classification errors. It is reported that when a suitable classification model has been developed for birth classification, decision support systems can be created to enable clinicians to gain in-depth insights into the patterns in the datasets. Developing such a system will not only allow health organizations to improve maternal health assessment processes, but also open doors for interdisciplinary research in two different fields in the region.Keywords
Cite This Article
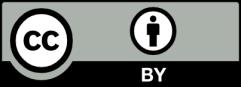
This work is licensed under a Creative Commons Attribution 4.0 International License , which permits unrestricted use, distribution, and reproduction in any medium, provided the original work is properly cited.