Open Access
ARTICLE
Analyzing the Impact of Scene Transitions on Indoor Camera Localization through Scene Change Detection in Real-Time
1 Department of Emergent Computing, School of Computing, Universiti Teknologi Malaysia, Johor Bahru, 81310, Malaysia
2 Malaysia-Japan International Institute of Technology (MJIIT), Universiti Teknologi Malaysia, Kuala Lumpur, 54100, Malaysia
3 Media and Game Innovation Centre of Excellence (MaGICX), Universiti Teknologi Malaysia, Johor Bahru, 81310, Malaysia
4 Department of Engineering Technology, University of Memphis, Memphis, TN, 38152, USA
5 Department of Computer Science and Artificial Intelligence, College of Computing and Information Technology, University of Bisha, Bisha, 61922, Saudi Arabia
6 School of Computing, Faculty of Engineering, Universiti Teknologi Malaysia, Johor Bahru, 81310, Malaysia
7 Department of Information System and Cyber Security, College of Computing and Information Technology, University of Bisha, Bisha, 61922, Saudi Arabia
* Corresponding Author: Muhammad S. Alam. Email:
Intelligent Automation & Soft Computing 2024, 39(3), 417-436. https://doi.org/10.32604/iasc.2024.051999
Received 20 March 2024; Accepted 25 April 2024; Issue published 11 July 2024
Abstract
Real-time indoor camera localization is a significant problem in indoor robot navigation and surveillance systems. The scene can change during the image sequence and plays a vital role in the localization performance of robotic applications in terms of accuracy and speed. This research proposed a real-time indoor camera localization system based on a recurrent neural network that detects scene change during the image sequence. An annotated image dataset trains the proposed system and predicts the camera pose in real-time. The system mainly improved the localization performance of indoor cameras by more accurately predicting the camera pose. It also recognizes the scene changes during the sequence and evaluates the effects of these changes. This system achieved high accuracy and real-time performance. The scene change detection process was performed using visual rhythm and the proposed recurrent deep architecture, which performed camera pose prediction and scene change impact evaluation. Overall, this study proposed a novel real-time localization system for indoor cameras that detects scene changes and shows how they affect localization performance.Keywords
Cite This Article
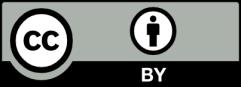