Open Access
ARTICLE
Intrusion Detection System for Smart Industrial Environments with Ensemble Feature Selection and Deep Convolutional Neural Networks
1 Dr. AHS Bukhari Postgraduate Centre of Information and Communication Technology, Faculty of Engineering and Technology, University of Sindh, Jamshoro, 76060, Pakistan
2 Newcastle Business School, Northumbria University, Newcastle, NE1, UK
* Corresponding Author: Asad Raza. Email:
Intelligent Automation & Soft Computing 2024, 39(3), 545-566. https://doi.org/10.32604/iasc.2024.051779
Received 15 March 2024; Accepted 10 May 2024; Issue published 11 July 2024
Abstract
Smart Industrial environments use the Industrial Internet of Things (IIoT) for their routine operations and transform their industrial operations with intelligent and driven approaches. However, IIoT devices are vulnerable to cyber threats and exploits due to their connectivity with the internet. Traditional signature-based IDS are effective in detecting known attacks, but they are unable to detect unknown emerging attacks. Therefore, there is the need for an IDS which can learn from data and detect new threats. Ensemble Machine Learning (ML) and individual Deep Learning (DL) based IDS have been developed, and these individual models achieved low accuracy; however, their performance can be improved with the ensemble stacking technique. In this paper, we have proposed a Deep Stacked Neural Network (DSNN) based IDS, which consists of two stacked Convolutional Neural Network (CNN) models as base learners and Extreme Gradient Boosting (XGB) as the meta learner. The proposed DSNN model was trained and evaluated with the next-generation dataset, TON_IoT. Several pre-processing techniques were applied to prepare a dataset for the model, including ensemble feature selection and the SMOTE technique. Accuracy, precision, recall, F1-score, and false positive rates were used to evaluate the performance of the proposed ensemble model. Our experimental results showed that the accuracy for binary classification is 99.61%, which is better than in the baseline individual DL and ML models. In addition, the model proposed for IDS has been compared with similar models. The proposed DSNN achieved better performance metrics than the other models. The proposed DSNN model will be used to develop enhanced IDS for threat mitigation in smart industrial environments.Keywords
Cite This Article
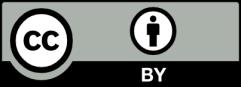
This work is licensed under a Creative Commons Attribution 4.0 International License , which permits unrestricted use, distribution, and reproduction in any medium, provided the original work is properly cited.