Open Access
ARTICLE
Systematic Cloud-Based Optimization: Twin-Fold Moth Flame Algorithm for VM Deployment and Load-Balancing
1 Information Science and Engineering College, Henan University of Technology, Zhengzhou, 450001, China
2 School of Artificial Intelligence and Big Data, Henan University of Technology, Zhengzhou, 450001, China
3 Key Laboratory of Grain Information Processing and Control, Ministry of Education, Henan University of Technology, Zhengzhou, 450001, China
* Corresponding Author: Tong Zhen. Email:
Intelligent Automation & Soft Computing 2024, 39(3), 477-510. https://doi.org/10.32604/iasc.2024.050726
Received 15 February 2024; Accepted 15 April 2024; Issue published 11 July 2024
Abstract
Cloud computing has gained significant recognition due to its ability to provide a broad range of online services and applications. Nevertheless, existing commercial cloud computing models demonstrate an appropriate design by concentrating computational assets, such as preservation and server infrastructure, in a limited number of large-scale worldwide data facilities. Optimizing the deployment of virtual machines (VMs) is crucial in this scenario to ensure system dependability, performance, and minimal latency. A significant barrier in the present scenario is the load distribution, particularly when striving for improved energy consumption in a hypothetical grid computing framework. This design employs load-balancing techniques to allocate different user workloads across several virtual machines. To address this challenge, we propose using the twin-fold moth flame technique, which serves as a very effective optimization technique. Developers intentionally designed the twin-fold moth flame method to consider various restrictions, including energy efficiency, lifespan analysis, and resource expenditures. It provides a thorough approach to evaluating total costs in the cloud computing environment. When assessing the efficacy of our suggested strategy, the study will analyze significant metrics such as energy efficiency, lifespan analysis, and resource expenditures. This investigation aims to enhance cloud computing techniques by developing a new optimization algorithm that considers multiple factors for effective virtual machine placement and load balancing. The proposed work demonstrates notable improvements of 12.15%, 10.68%, 8.70%, 13.29%, 18.46%, and 33.39% for 40 count data of nodes using the artificial bee colony-bat algorithm, ant colony optimization, crow search algorithm, krill herd, whale optimization genetic algorithm, and improved Lévy-based whale optimization algorithm, respectively.Keywords
Cite This Article
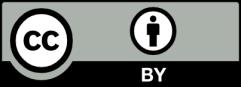