Open Access
ARTICLE
Improving VQA via Dual-Level Feature Embedding Network
School of Computer Engineering and Science, Shanghai University, Shanghai, 200444, China
* Corresponding Author: Yaru Song. Email:
Intelligent Automation & Soft Computing 2024, 39(3), 397-416. https://doi.org/10.32604/iasc.2023.040521
Received 21 March 2023; Accepted 08 June 2023; Issue published 11 July 2024
Abstract
Visual Question Answering (VQA) has sparked widespread interest as a crucial task in integrating vision and language. VQA primarily uses attention mechanisms to effectively answer questions to associate relevant visual regions with input questions. The detection-based features extracted by the object detection network aim to acquire the visual attention distribution on a predetermined detection frame and provide object-level insights to answer questions about foreground objects more effectively. However, it cannot answer the question about the background forms without detection boxes due to the lack of fine-grained details, which is the advantage of grid-based features. In this paper, we propose a Dual-Level Feature Embedding (DLFE) network, which effectively integrates grid-based and detection-based image features in a unified architecture to realize the complementary advantages of both features. Specifically, in DLFE, In DLFE, firstly, a novel Dual-Level Self-Attention (DLSA) modular is proposed to mine the intrinsic properties of the two features, where Positional Relation Attention (PRA) is designed to model the position information. Then, we propose a Feature Fusion Attention (FFA) to address the semantic noise caused by the fusion of two features and construct an alignment graph to enhance and align the grid and detection features. Finally, we use co-attention to learn the interactive features of the image and question and answer questions more accurately. Our method has significantly improved compared to the baseline, increasing accuracy from 66.01% to 70.63% on the test-std dataset of VQA 1.0 and from 66.24% to 70.91% for the test-std dataset of VQA 2.0.Keywords
Cite This Article
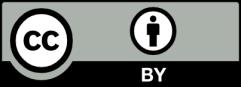
This work is licensed under a Creative Commons Attribution 4.0 International License , which permits unrestricted use, distribution, and reproduction in any medium, provided the original work is properly cited.