Open Access
ARTICLE
Chaotic Elephant Herd Optimization with Machine Learning for Arabic Hate Speech Detection
1 Department of Language Preparation, Arabic Language Teaching Institute, Princess Nourah bint Abdulrahman University, P.O. Box 84428, Riyadh, 11671, Saudi Arabia
2 Department of Industrial Engineering, College of Engineering at Alqunfudah, Umm Al-Qura University, Mecca, 24382, Saudi Arabia
3 Prince Saud AlFaisal Institute for Diplomatic Studies, Riyadh, 12735, Saudi Arabia
4 Department of Computer Science, College of Computing and Information Technology, Shaqra University, Shaqra, 11911, Saudi Arabia
5 Department of Information Systems, College of Computer and Information Sciences, Princess Nourah bint Abdulrahman University, P.O. Box 84428, Riyadh, 11671, Saudi Arabia
6 Department of Computer Science, Faculty of Computers and Information Technology, Future University in Egypt New Cairo, New Cairo, 11835, Egypt
7 Department of Computer and Self Development, Preparatory Year Deanship, Al-Kharj, 16278, Saudi Arabia
* Corresponding Author: Abdelwahed Motwakel. Email:
Intelligent Automation & Soft Computing 2024, 39(3), 567-583. https://doi.org/10.32604/iasc.2023.033835
Received 29 June 2022; Accepted 14 October 2022; Issue published 11 July 2024
Abstract
In recent years, the usage of social networking sites has considerably increased in the Arab world. It has empowered individuals to express their opinions, especially in politics. Furthermore, various organizations that operate in the Arab countries have embraced social media in their day-to-day business activities at different scales. This is attributed to business owners’ understanding of social media’s importance for business development. However, the Arabic morphology is too complicated to understand due to the availability of nearly 10,000 roots and more than 900 patterns that act as the basis for verbs and nouns. Hate speech over online social networking sites turns out to be a worldwide issue that reduces the cohesion of civil societies. In this background, the current study develops a Chaotic Elephant Herd Optimization with Machine Learning for Hate Speech Detection (CEHOML-HSD) model in the context of the Arabic language. The presented CEHOML-HSD model majorly concentrates on identifying and categorising the Arabic text into hate speech and normal. To attain this, the CEHOML-HSD model follows different sub-processes as discussed herewith. At the initial stage, the CEHOML-HSD model undergoes data pre-processing with the help of the TF-IDF vectorizer. Secondly, the Support Vector Machine (SVM) model is utilized to detect and classify the hate speech texts made in the Arabic language. Lastly, the CEHO approach is employed for fine-tuning the parameters involved in SVM. This CEHO approach is developed by combining the chaotic functions with the classical EHO algorithm. The design of the CEHO algorithm for parameter tuning shows the novelty of the work. A widespread experimental analysis was executed to validate the enhanced performance of the proposed CEHOML-HSD approach. The comparative study outcomes established the supremacy of the proposed CEHOML-HSD model over other approaches.Keywords
Cite This Article
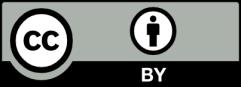
This work is licensed under a Creative Commons Attribution 4.0 International License , which permits unrestricted use, distribution, and reproduction in any medium, provided the original work is properly cited.