Open Access
ARTICLE
Multi-Scale Location Attention Model for Spatio-Temporal Prediction of Disease Incidence
1 College of Computer, National University of Defense Technology, Changsha, 410073, China
2 Technical Service Center for Vocational Education, National University of Defense Technology, Changsha, 410073, China
3 School of Computing and Communications, Lancaster University, Lancaster, LA1 4WA, UK
* Corresponding Author: Zhiping Cai. Email:
Intelligent Automation & Soft Computing 2024, 39(3), 585-597. https://doi.org/10.32604/iasc.2023.030221
Received 21 March 2022; Accepted 15 June 2022; Issue published 11 July 2024
Abstract
Due to the increasingly severe challenges brought by various epidemic diseases, people urgently need intelligent outbreak trend prediction. Predicting disease onset is very important to assist decision-making. Most of the existing work fails to make full use of the temporal and spatial characteristics of epidemics, and also relies on multivariate data for prediction. In this paper, we propose a Multi-Scale Location Attention Graph Neural Networks (MSLAGNN) based on a large number of Centers for Disease Control and Prevention (CDC) patient electronic medical records research sequence source data sets. In order to understand the geography and timeliness of infectious diseases, specific neural networks are used to extract the geography and timeliness of infectious diseases. In the model framework, the features of different periods are extracted by a multi-scale convolution module. At the same time, the propagation effects between regions are simulated by graph convolution and attention mechanisms. We compare the proposed method with the most advanced statistical methods and deep learning models. Meanwhile, we conduct comparative experiments on data sets with different time lengths to observe the prediction performance of the model in the face of different degrees of data collection. We conduct extensive experiments on real-world epidemic-related data sets. The method has strong prediction performance and can be readily used for epidemic prediction.Keywords
Cite This Article
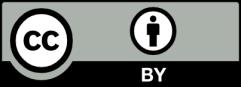