Open Access
ARTICLE
A Hybrid Manufacturing Process Monitoring Method Using Stacked Gated Recurrent Unit and Random Forest
1 Department of Industrial Management, National Taiwan University of Science and Technology, Taipei, Taiwan
2 Faculty of Mechanical and Industrial Engineering, Bahir Dar Institute of Technology (BiT), Bahir Dar University, Bahir Dar, 6000, Ethiopia
3 Department of Computer Science and Information Engineering, National Taiwan University of Science and Technology, Taipei, Taiwan
4 Department of Information System, Faculty of Engineering and Computer Science, Krida Wacana Christian University, Jakarta, Indonesia
5 Department of Information Management, National Dong Hwa University, Hualien, Taiwan
* Corresponding Author: Chao-Lung Yang. Email:
(This article belongs to the Special Issue: Artificial Intelligence Algorithm for Industrial Operation Application)
Intelligent Automation & Soft Computing 2024, 39(2), 233-254. https://doi.org/10.32604/iasc.2024.043091
Received 21 June 2023; Accepted 23 January 2024; Issue published 21 May 2024
Abstract
This study proposed a new real-time manufacturing process monitoring method to monitor and detect process shifts in manufacturing operations. Since real-time production process monitoring is critical in today’s smart manufacturing. The more robust the monitoring model, the more reliable a process is to be under control. In the past, many researchers have developed real-time monitoring methods to detect process shifts early. However, these methods have limitations in detecting process shifts as quickly as possible and handling various data volumes and varieties. In this paper, a robust monitoring model combining Gated Recurrent Unit (GRU) and Random Forest (RF) with Real-Time Contrast (RTC) called GRU-RF-RTC was proposed to detect process shifts rapidly. The effectiveness of the proposed GRU-RF-RTC model is first evaluated using multivariate normal and non-normal distribution datasets. Then, to prove the applicability of the proposed model in a real manufacturing setting, the model was evaluated using real-world normal and non-normal problems. The results demonstrate that the proposed GRU-RF-RTC outperforms other methods in detecting process shifts quickly with the lowest average out-of-control run length (ARL) in all synthesis and real-world problems under normal and non-normal cases. The experiment results on real-world problems highlight the significance of the proposed GRU-RF-RTC model in modern manufacturing process monitoring applications. The result reveals that the proposed method improves the shift detection capability by 42.14% in normal and 43.64% in gamma distribution problems.Keywords
Cite This Article
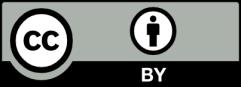
This work is licensed under a Creative Commons Attribution 4.0 International License , which permits unrestricted use, distribution, and reproduction in any medium, provided the original work is properly cited.