Open Access
ARTICLE
A Reference Vector-Assisted Many-Objective Optimization Algorithm with Adaptive Niche Dominance Relation
1 School of Computer Science and Technology, Huaibei Normal University, Huaibei, 340604, China
2 School of Physic and Electronic Information, Huaibei Normal University, Huaibei, 340604, China
3 Anhui Engineering Research Center for Intelligent Computing and Application on Cognitive Behavior (ICACB), Huaibei Normal University, Huaibei, 340604, China
4 Anhui Province Key Laboratory of Intelligent Computing and Applications, Huaibei Normal University, Huaibei, 340604, China
5 Institute of Artificial Intelligence, Hefei Comprehensive National Science Center, Hefei, 230000, China
* Corresponding Author: Yating Wu. Email:
(This article belongs to the Special Issue: Artificial Intelligence Algorithm for Industrial Operation Application)
Intelligent Automation & Soft Computing 2024, 39(2), 189-211. https://doi.org/10.32604/iasc.2024.042841
Received 14 June 2023; Accepted 31 January 2024; Issue published 21 May 2024
Abstract
It is still a huge challenge for traditional Pareto-dominated many-objective optimization algorithms to solve many-objective optimization problems because these algorithms hardly maintain the balance between convergence and diversity and can only find a group of solutions focused on a small area on the Pareto front, resulting in poor performance of those algorithms. For this reason, we propose a reference vector-assisted algorithm with an adaptive niche dominance relation, for short MaOEA-AR. The new dominance relation forms a niche based on the angle between candidate solutions. By comparing these solutions, the solution with the best convergence is found to be the non-dominated solution to improve the selection pressure. In reproduction, a mutation strategy of -bit crossover and hybrid mutation is used to generate high-quality offspring. On 23 test problems with up to 15-objective, we compared the proposed algorithm with five state-of-the-art algorithms. The experimental results verified that the proposed algorithm is competitive.Keywords
Supplementary Material
Supplementary Material FileMany problems in practical applications can be seen as multi-objective optimization problems (MOPs), such as cloud computing [1], industrial copper burdening system [2], and engineering design [3,4]. Many-objective optimization problem (MaOP) [5] is a task that needs to simultaneously optimize
where
Owing to the strong population-based search properties of multi-objective evolutionary algorithms (MOEAs), they can be solved efficiently for MOPs. However, when encountering MaOPs, the performance of MOEA will deteriorate rapidly due to the curse of dimensional [6]. Researchers have developed new algorithms to solve MaOPs. It can be classified into four types: Convergence enhancement, Decomposition-based, Indicator-based and Secondary selection criteria.
The first category includes various convergence enhancement methods. The convergence pressure loss of most traditional MOEAs is the direct result of the inability to distinguish the traditional dominance relation. The most direct convergence enhancement method is to modify the dominance relation to improve the selection pressure. For example,
The second category refers to Decomposition-based algorithms that decompose the MOP into multiple single-objective optimization problems. For example, the Multi-objective evolutionary algorithm based on decomposition (MOEA/D) [9] proposed by Zhang and Li decomposes
The third category is indicator-based methods [12], which proposes a number of MOEAs based on hypervolume (HV). Their main disadvantage is the high computational complexity of HV, especially when solving the MaOP. Sun et al. [13] introduced an Inverse Generation Distance (IGD) indicator in MOEA to solve the MaOP with up to 20-objective. An evolutionary algorithm (IGD+EMOA) based on inverse generation distance plus (IGD+) indicator was proposed [14] to solve the MaOP with up to 8-objective. The IGD+ indicator is a variant of the IGD.
The fourth category is characterized by a combination of Pareto dominance and other selection criteria. These methods choose high ranking non-dominated solutions based on Pareto dominance first, and then use the secondary selection criterion to distinguish non-comparable candidates. Existing methods in this genre mainly employ three typical ideas. The first is to develop new criteria to support methods with good convergence and diversity in non-comparable candidate solutions, such as Promising-region based EMO algorithm (PREA) [15]. The second idea is to use performance indicators to distinguish the quality of non-comparable candidate solutions by selecting those with great contributions, such as the Kriging-assisted Two_Arch2 (KTA-2) [16]. The typical algorithm of the third idea is Many-objective evolutionary algorithm based on dominance and decomposition (MOEA/DD) [17], which combines Pareto dominance with decomposition-based algorithms.
The existing four classes of methods have been able to solve many-objective optimization problems well, but there are still various shortcomings. Decomposition-based algorithms are seriously affected by the weight vector setting, and the computational complexity of indicator-based algorithms is high. Most MaOEAs mainly improve the performance of the algorithm from the perspective of environmental selection, and little attention has been paid to offspring generation. In addition, as far as most dominance relations are concerned, some methods can easily generate the solution set concentrated in a small region of the Pareto front. Considering these issues, in this paper, we propose a reference vector-assisted many-objective optimization algorithm with adaptive niche dominance relations (MaOEA-AR). First using
1. A new dominance relation, called the adaptive niche dominance relation (A-NDR), is proposed for many-objective optimization. By developing niche techniques based on the angle between candidate solutions and having just one candidate solution with the best convergence in each niche, A-NDR balances convergence and diversity, where convergence is calculated in terms of the PF shape adaptively by selecting the convergence indicator. The proposed A-NDR is parameter-free since the size of the niche is determined adaptively by the distribution of candidate solutions.
2. Mating selection also uses the niche mating method to select neighbor solutions in the objective space, the
The rest of this paper is structured as follows. The proposed algorithm is further explained in Section 2. The experimental design and some of the data analysis are presented in Section 3. Finally, Section 4 presents the conclusions and outlook for the future. The related works, motivation, some of the experimental results and analyses of this paper are given in the Supplementary Material.
This section describes the proposed algorithm MaOEA-AR in detail. First, the general framework of the algorithm is given. The reproduction strategy was then discussed. Subsequently, we describe the environment selection, as well as the adaptive niche dominance relation and angle penalty distance used in the environment selection.
The details of the general framework of the proposed MaOEA-AR are presented in Algorithm 1. First, initialize (lines 1–3) to generate a set of uniformly distributed reference vectors, initial populations, and train the initial improved growing neural gas (iGNG) net [20] using reference vectors with associated solutions. Copy the non-dominant solution from the initial population into archive
As shown in Algorithm 2, in lines 1–12, the niche mating randomly selects 80% or 20% of the parent individuals from local neighbors or whole populations, which is similar to the traditional setting [21], to avoid reproducing the same offspring as the parents. Niche is a more stable and effect way to exchange evolutionary information between two solutions with similar objective vectors. In line 3, the solution is selected by normalizing the objective values to the same range to ensure fairness. In lines 13–20,
Algorithm 3 describes the hybrid mutation method in detail, in which the mutation and the traditional mutation are randomly applied at a set ratio of 20% and 80%, respectively. We have no attempt to find the best ratio, but the probability of using the traditional bit-wise mutation method should be set relatively larger, because it provides a more general and stable variation. It is worth noting that
The environmental selection process is presented in Algorithm 4. The environmental selection consisted of three main parts. The first part combined the populations and archives for selection. The second is to perform an adaptive niche dominance relation ranking to locate key layers. The third is the APD selection, which is the same as in [19]. Here, we use the adaptive niche dominance relation to identify non-dominated solutions in the population and remove extreme solutions with a large Euclidean distances from the ideal point. The number of solutions in the population after APD selection may be greater than
2.3.1 Adaptive Niche Dominance Relation
Inspired by the literature [22], we propose an adaptive niche dominance relation (A-NDR) to balance the convergence and diversity of non-dominated solutions using the niche technique in this study. Specifically, in the A-NDR, if and only if
where
and
Figure 1: Describes an example of PF shape estimation. Given three solutions
It can be seen from Eq. (5) that each solution in the population has a niche of size
Figure 2: Dominant region of the solution obtained by A-NDR in bi-objective spaces
2.3.2 Angle Penalized Distance
The objective function is converted to the same range by using Eq. (6).
divide population
Figure 3: Individual and reference vector association diagram
The relation between the two sub-spaces is measured by the cosine value of the acute angle.
where
where
and
where
MaOEA-AR consists of three main components: (1) Reproduction (
In this section, we experiments on 23 benchmark test problems from three widely used test suites (i.e., Benchmark MOP proposed by Deb, Thiele, Laumanns, and Zitzler (DTLZ) [25], MaF [26] and Benchmark MOP proposed by Walking Fish Group (WFG) [27]) to compare MaOEA-AR with five state-of-the-art algorithms (i.e., Many-objective evolutionary algorithms based on an independent two-stage (MaOEA-IT) [28], IGD based many-objective evolutionary algorithm (MaOEA-IGD) [13], Many-objective evolutionary algorithm based on objective space reduction (MaOEA-RD) [29], MOEA/D with update when required (MOEA/D-UR) [30] and MOEA/D [9]). We chose the commonly used comprehensive evaluation indicators HV [31] and IGD [32]. The experiments were conducted on Evolutionary multi-objective optimization platform (PlatEMO) [33], and the comparison algorithm was obtained from the platform.
On the 23 benchmark problems of DTLZ, MaF and WFG test suite, we compared the proposed algorithm with the five state-of-the-art algorithms. All problems in the DTLZ and WFG test suites can have any set of decision variable dimensions and objective numbers. MaF was proposed by Cheng et al. [26] in 2017 and includes various representative problems in the real-world. Its test suite contains 15 multi-objective optimization problems chosen by MaF 1–7 to evaluate the algorithm’s performance in solving complex problems. Some properties of these benchmark function suites are listed in Table 1.
With the continuous proposal of MaOEAs, evaluating the performance of MaOEA has also become an important research direction. When the algorithms solve MaOPs, it is necessary to quantify the performance of the algorithm through performance indicators, and to quantify the performance of one algorithm in comparison with the performance of other algorithms. The comprehensive indicators HV and IGD are the most often utilized indicators. Based on the magnitude of the range covered by the PS computed by the algorithm in the objective space, HV is used to evaluate convergence and diversity. By evaluating the similarity between the true PF and the PF generated by the algorithm, the IGD evaluates convergence and diversity. In general, the algorithm performs better the lower the IGD value, whereas the opposite is true for HV. In this study, we use these two indicators to evaluate the performance of the proposed MaOEA-AR, as shown in Eqs. (11) and (12).
where PF is obtained by algorithm,
Population size
Numbers of run and stop conditions: To obtain reliable experimental results, we ran each algorithm 20 times independently on each test instance. This end when the maximum number of function evaluations (maxFEs) is reached. To ensure that each algorithm converges, we uniformly set the maxFEs at 100,000 for each test instance.
Operator parameter settings: The crossover probability
Two parameters in MaOEA-AR require to be predefined, i.e., the user-defined parameter
3.4 Experimental Results Analysis
In this section, we analyze and evaluate the MaOEA-AR based on the statistical results of 20 independent runs of each algorithm on 16 benchmark problems (92 test instances). Wilcoxon rank sum test is used to compare the difference between the average value obtained by MaOEA-AR and the other five algorithms. The significance level was 0.05, and the ‘+’, ‘−’ and ‘=’ in the table indicate that the performance of other algorithms is significantly better, significantly lower, and no different than MaOEA-AR. Table 3 exhibits the performance of MaOEA-AR on 23 benchmark problems with five comparison algorithms, and the Wilcoxon rank-sum test of MaOEA-AR on the test instances is shown in Fig. 4. It can be seen that MaOEA-AR has strong competitiveness for both HV and IGD.
Figure 4: Wilcoxon rank sum test of MaOEA-AR on test instances in terms of HV and IGD
In this paper we conducted 5 groups of experiments to verify the performance of the algorithm and the effectiveness of each strategy. We conducted experiments on all three benchmark test suites, DTLZ, MaF and WFG. The results and analyses of the first group of DTLZ benchmark experiments and the second group of MaF benchmark experiments are given in the Supplementary Material, and the results and analyses of the third group of WFG benchmark experiments are given below. The 4th group of experiments are ablation experiments to validate the performance of the A-NDR and
The experimental statistics of the six algorithms on the WFG test problem are given in Tables 4 and 5. MaOEA-AR has performed very competitively on most problems. In Table 4, we can see that WFG2 is a difficult problem, where PF is disconnected. Similar to other algorithms, MaOEA-AR does not handle this problem well, but MOEA/D-UR can solve the WFG2 problem well and obtain the best performance on its objectives in any dimension. This is because the decomposition-based algorithm predefines a set of well-distributed weight vectors that improve the diversity of the solutions obtained, in addition, the algorithm uses a specific mechanism to maintain a good balance between diversity and convergence. MaOEA-AR obtained 23 optimal values on 36 test problems, and optimal values on any dimension of the objective in WFG4, WFG5, WFG7 and WFG9. MOEA/D-UR followed closely behind, obtaining 11 optimal values. Only two optimal values were obtained for MaOEA-IGD. MaOEA-IT, MaOEA-RD and MOEA/D did not obtain optimal values. There are several reasons for this phenomenon: First, at mating selection,
Figure 5: Distribution of solutions on WFG9 with 15-objective
Figure 6: Distribution of solutions on WFG9 with 3-objective
To observe the evolution of the algorithms, the evolutionary trajectory of the IGD values of the experimental results obtained using the five state-of-the-art algorithms and MaOEA-AR on the 10-objectives DTLZ4, WFG4 and WFG9 are shown in Fig. 7. In these plots, the X-axis denotes the number of functional assessments, and the Y-axis denotes the value of the IGD indicator, with the data used for plotting obtained from the data closest to the average IGD value. Throughout the evolutionary process, MaOEA-AR significantly outperformed other algorithms on the DTLZ4, WFG4 and WFG9 problems, which makes it competitive in terms of the balance between convergence and diversity. It is clear from Fig. 7 that MaOEA-AR all converge very quickly and are more robust than the other five algorithms. MaOEA-AR converges steadily and rapidly during the evolution. This is because the population itself dynamically guides its evolution towards PF, and A-NDR accelerates its convergence.
Figure 7: Convergence curves of the algorithms IGD on DTLZ4, WFG4 and WFG9 with 10-objective
3.4.2 Performance on Real-World Applications
To verify the algorithm’s ability to solve real-world optimization problems, three of the many-objective test problems from the RE test suite were selected to validate MaOEA-AR’s ability to solve real-world problems. The RE test suite consists of 16 real-world problems with bounded constraints, we have chosen among them RE4-7-1, RE4-6-2 and RE6-3-1 for experiments, whose corresponding real-world problems and their characteristics are given in Table 6. We refer to the settings in [34], where the population size is 120 when
The above experimental results show that MaOEA-AR has strong competitiveness in these conventional problems and some real-world representative problems. This can be explained by the fact that our adaptive niche dominance relation is different from the traditional Pareto dominance relations and the existing improved dominance. Most dominance relations can only find a set of solutions concentrated in a small area on the Pareto front. In this study, we used a customized niche technique to balance the diversity of non-dominated convergence, which generates selection pressure even in the high-dimensional objective space, and comparability between non-dominated solutions, alleviating the dominance resistance phenomenon. In the process of generating offspring, we used
In this study, we proposed a reference vector-assisted algorithm MaOEA-AR with an adaptive niche dominance relation to solve MaOPs. We balance the convergence and diversity of the non-dominated solution set by a customized niche technique, where the convergence indicator is determined by the ratio of the distance from the ideal point to the solution and the distance from the ideal point to the hyperplane to select the appropriate convergence indicator by determining the PF shape to maximize the selection pressure of the algorithm. When reproducing offspring are generated,
Acknowledgement: We thank PlatEMO, an evolutionary multi-objective optimization platform developed by BIMK group at Anhui University, for providing us with a good experimental environment.
Funding Statement: This research is supported by the National Natural Science Foundation of China (Grant No. 61976101), the University Natural Science Research Project of Anhui Province (Grant No. 2023AH040056), the Natural Science Research Project of Anhui Province (Graduate Research Project, Grant No. YJS20210463), the Funding Plan for Scientic Research Activities of Academic and Technical Leaders and Reserve Candidates in Anhui Province (Grant No. 2021H264), the Top Talent Project of Disciplines (Majors) in Colleges and Universities in Anhui Province (Grant No. gxbjZD2022021), the University Synergy Innovation Program of Anhui Province, China (GXXT-2022-033) and supported by the Innovation Fund for Postgraduates of Huaibei Normal University (Grant Nos. cx2022041, yx2021023, CX2023043). We would like to thank Editage (www.editage.cn) for English language editing.
Author Contributions: The authors confirm contribution to the paper as follows: Conceptualization of this study, Methodology, Software, Writing: Yating Wu; Supervision, Writing-review & editing, Funding acquisition: Fangzhen Ge; Visualization, Investigation: Debao Chen; Supervision: Longfeng Shen. All authors reviewed the results and approved the final version of the manuscript.
Availability of Data and Materials: The experiment in this paper is a simulation experiment, which is completed on the evolutionary multi-objective optimization platform PlatEMO. The comparison algorithm and test function are all from this platform. The experimental data can be found in this paper and Supplementary Material.
Conflicts of Interest: The authors declare that they have no conflicts of interest to report regarding the present study.
Supplementary Materials: The supplementary material is available online at https://doi.org/10.32604/iasc.2024.042841.
References
1. J. L. Xu, Z. X. Zhang, Z. M. Hu, L. Du, and X. J. Cai, “A many-objective optimized task allocation scheduling model in cloud computing,” Appl. Intell., vol. 51, no. 6, pp. 3293–3310, 2021. doi: 10.1007/s10489-022-03963-w. [Google Scholar] [CrossRef]
2. L. B. Ma et al., “Learning to optimize: Reference vector reinforcement learning adaption to constrained many-objective optimization of industrial copper burdening system,” IEEE Trans. Cybern., vol. 52, no. 12, pp. 12698–12711, Dec. 2022. doi: 10.1109/TCYB.2021.3086501 [Google Scholar] [PubMed] [CrossRef]
3. Z. Y. Hu, Z. H. Wei, H. Sun, J. M. Yang, and L. X. Wei, “Optimization of metal rolling control using soft computing approaches: A review,” Arch. Comput. Method Eng., vol. 28, no. 2, pp. 405–421, 2021. doi: 10.1007/s11831-018-9250-y. [Google Scholar] [CrossRef]
4. X. F. Liu, Z. H. Zhan, and J. Zhang, “Resource-aware distributed differential evolution for training expensive neural-network-based controller in power electronic circuit,” IEEE Trans. Neural Netw. Learn. Syst., vol. 33, no. 11, pp. 6286–6296, 2022. doi: 10.1109/TNNLS.2021.3075205 [Google Scholar] [PubMed] [CrossRef]
5. M. Farina and P. Amato, “On the optimal solution definition for many-criteria optimization problems,” in 2002 Annu. Meet. North Am. Fuzzy Inf. Process. Soc. Proc. NAFIPS-FLINT 2002 (Cat. No. 02TH8622), New Orleans, LA, USA, 2002, pp. 233–238. [Google Scholar]
6. R. C. Purshouse and P. J. Fleming, “On the evolutionary optimization of many conflicting objectives,” IEEE Trans. Evolut. Comput., vol. 11, no. 6, pp. 770–784, 2007. doi: 10.1109/TEVC.2007.910138. [Google Scholar] [CrossRef]
7. M. Laumanns, L. Thiele, K. Deb, and E. Zitzler, “Combining convergence and diversity in evolutionary multi-objective optimization,” Evol. Comput., vol. 10, no. 3, pp. 263–282, 2002. doi: 10.1162/106365602760234108 [Google Scholar] [PubMed] [CrossRef]
8. S. X. Yang, M. Q. Li, X. H. Liu, and J. H. Zheng, “A grid-based evolutionary algorithm for many-objective optimization,” IEEE Trans. Evolut. Comput., vol. 17, no. 5, pp. 721–736, 2013. doi: 10.1109/TEVC.2012.2227145. [Google Scholar] [CrossRef]
9. Q. F. Zhang and H. Li, “MOEA/D: A multi-objective evolutionary algorithm based on decomposition,” IEEE Trans. Evolut. Comput., vol. 11, no. 6, pp. 712–731, 2007. doi: 10.1109/TEVC.2007.892759. [Google Scholar] [CrossRef]
10. H. L. Liu, F. Q. Gu, and Q. F. Zhang, “Decomposition of a multi-objective optimization problem into a number of simple multi-objective sub-problems,” IEEE Trans. Evolut. Comput., vol. 18, no. 3, pp. 450–455, 2014. doi: 10.1109/TEVC.2013.2281533. [Google Scholar] [CrossRef]
11. L. B. Ma, M. Huang, S. X. Yang, R. Wang, and X. W. Wang, “An adaptive localized decision variable analysis approach to large-scale multi-objective and many-objective optimization,” IEEE Trans. Cybern., vol. 52, no. 7, pp. 6684–6696, Jul. 2022. doi: 10.1109/TCYB.2020.3041212 [Google Scholar] [PubMed] [CrossRef]
12. D. Brockhoff and E. Zitzler, “Improving hypervolume-based multi-objective evolutionary algorithms by using objective reduction methods,” in 2007 IEEE Congr. Evol. Comput., Singapore, 2007, pp. 2086–2093. [Google Scholar]
13. Y. N. Sun, G. G. Yen, and Y. Zhang, “IGD indicator-based evolutionary algorithm for many-objective optimization problems,” IEEE Trans. Evolut. Comput., vol. 23, no. 2, pp. 173–187, 2019. doi: 10.1109/TEVC.2018.2791283. [Google Scholar] [CrossRef]
14. E. M. Lopez and C. A. C. Coello, “IGD+-EMOA: A multi-objective evolutionary algorithm based on IGD+,” in 2016 IEEE Congr. Evol. Comput., Vancouver, BC, Canada, 2016, pp. 999–1006. [Google Scholar]
15. J. W. Yuan, H. L. Liu, F. Q. Gu, Q. F. Zhang, and Z. S. He, “Investigating the properties of indicators and an evolutionary many-objective algorithm using promising regions,” IEEE Trans. Evolut. Comput., vol. 25, no. 1, pp. 75–86, 2021. doi: 10.1109/TEVC.2020.2999100. [Google Scholar] [CrossRef]
16. Z. S. Song, H. D. Wang, C. He, and Y. C. Jin, “A kriging-assisted two-archive evolutionary algorithm for expensive many-objective optimization,” IEEE Trans. Evolut. Comput., vol. 25, no. 6, pp. 1013–1027, 2021. doi: 10.1109/TEVC.2021.3073648. [Google Scholar] [CrossRef]
17. K. Li, K. Deb, Q. F. Zhang, and S. Kwong, “An evolutionary many-objective optimization algorithm based on dominance and decomposition,” IEEE Trans. Evolut. Comput., vol. 19, no. 5, pp. 694–716, 2015. doi: 10.1016/j.knosys.2021.107392. [Google Scholar] [CrossRef]
18. H. Xu, B. Xue, and M. J. Zhang, “A duplication analysis-based evolutionary algorithm for bi-objective feature selection,” IEEE Trans. Evolut. Comput., vol. 2, no. 2, pp. 205–218, 2021. doi: 10.1109/TEVC.2020.3016049. [Google Scholar] [CrossRef]
19. R. Cheng, Y. C. Jin, M. Olhofer, and B. Sendhoff, “A reference vector guided evolutionary algorithm for many-objective optimization,” IEEE Trans. Evolut. Comput., vol. 20, no. 5, pp. 773–791, 2016. doi: 10.1109/TEVC.2016.2519378. [Google Scholar] [CrossRef]
20. Q. Q. Liu, Y. C. Jin, M. Heiderich, T. Rodemann, and Y. Guo, “An adaptive reference vector-guided evolutionary algorithm using growing neural gas for many-objective optimization of irregular problems,” IEEE Trans. Cybern., vol. 52, no. 5, pp. 2698–2711, 2022. doi: 10.1109/TCYB.2020.3020630 [Google Scholar] [PubMed] [CrossRef]
21. B. H. Nguyen, B. Xue, P. Andreae, H. Ishibuchi, and M. J. Zhang, “Multiple reference points-based decomposition for multi-objective feature selection in classification: Static and dynamic mechanisms,” IEEE Trans. Evolut. Comput., vol. 24, no. 1, pp. 170–184, 2020. doi: 10.1109/TEVC.2019.2913831. [Google Scholar] [CrossRef]
22. Y. Tian, R. Cheng, X. Y. Zhang, Y. S. Su, and Y. C. Jin, “A strengthened dominance relation considering convergence and diversity for evolutionary many-objective optimization,” IEEE Trans. Evolut. Comput., vol. 23, no. 2, pp. 331–345, 2019. doi: 10.1109/TEVC.2018.2866854. [Google Scholar] [CrossRef]
23. L. Li, G. G. Yen, A. Sahoo, L. Chang, and T. Gu, “On the estimation of pareto front and dimensional similarity in many-objective evolutionary algorithm,” Inform. Sci., vol. 563, pp. 375–400, 2021. doi: 10.1016/j.ins.2021.03.008. [Google Scholar] [CrossRef]
24. K. Deb and H. Jain, “An evolutionary many-objective optimization algorithm using reference-point-based non-dominated sorting approach, part I: Solving problems with box constraints,” IEEE Trans. Evolut. Comput., vol. 18, no. 4, pp. 577–601, 2014. doi: 10.1109/TEVC.2013.2281535. [Google Scholar] [CrossRef]
25. K. Deb, L. Thiele, M. Laumanns, and E. Zitzler, “Scalable test problems for evolutionary multi-objective optimization,” in Proc. 2002 Congr. Evol. Comput. (Cat. No.02TH8600), Singapore, 2002, pp. 105–145. [Google Scholar]
26. R. Cheng et al., “A benchmark test suite for evolutionary many-objective optimization,” Complex Intell. Syst., vol. 3, no. 1, pp. 67–81, 2017. [Google Scholar]
27. S. Huband, P. Hingston, L. Barone, and L. While, “A review of multi-objective test problems and a scalable test problem toolkit,” IEEE Trans. Evolut. Comput., vol. 10, no. 5, pp. 477–506, 2006. doi: 10.1109/TEVC.2005.861417. [Google Scholar] [CrossRef]
28. Y. N. Sun, B. Xue, M. J. Zhang, and G. G. Yen, “A new two-stage evolutionary algorithm for many-objective optimization,” IEEE Trans. Evolut. Comput., vol. 23, no. 5, pp. 748–761, 2019. doi: 10.1109/TEVC.2018.2882166. [Google Scholar] [CrossRef]
29. Z. He and G. G. Yen, “Many-objective evolutionary algorithm: Objective space reduction and diversity improvement,” IEEE Trans. Evolut. Comput., vol. 20, no. 1, pp. 145–160, 2016. doi: 10.1109/TEVC.2015.2433266. [Google Scholar] [CrossRef]
30. L. R. C. de Farias and A. F. R. Araujo, “A decomposition-based many-objective evolutionary algorithm updating weights when required,” Swarm and Evolutionary Computation. vol. 68. pp. 100980, Feb. 2022. [Google Scholar]
31. L. While, P. Hingston, L. Barone, and S. Huband, “A faster algorithm for calculating hypervolume,” IEEE Trans. Evolut. Comput., vol. 10, no. 1, pp. 29–38, 2006. doi: 10.1109/TEVC.2005.851275. [Google Scholar] [CrossRef]
32. E. Zitzler, L. Thiele, and M. Laumanns, “Performance assessment of multi-objective optimizers: An analysis and review,” IEEE Trans. Evolut. Comput., vol. 7, no. 2, pp. 117–132, 2003. doi: 10.1109/TEVC.2003.810758. [Google Scholar] [CrossRef]
33. Y. Tian, R. Cheng, X. Y. Zhang, and Y. C. Jin, “PlatEMO: A MATLAB platform for evolutionary multi-objective optimization [educational forum],” IEEE Comput. Intell. Mag., vol. 12, no. 4, pp. 73–87, 2017. doi: 10.1109/MCI.2017.2742868. [Google Scholar] [CrossRef]
34. R. Tanabe and H. Ishibuchi, “An easy-to-use real-world multi-objective optimization problem suite,” Appl. Soft. Comput., vol. 89, pp. 106078, Apr. 2020. doi: 10.1016/j.asoc.2020.106078. [Google Scholar] [CrossRef]
35. Q. L. Zhu et al., “An elite gene guided reproduction operator for many-objective optimization,” IEEE Trans. Cybern., vol. 51, no. 2, pp. 765–778, 2021. doi: 10.1109/TCYB.2019.2932451 [Google Scholar] [PubMed] [CrossRef]
36. H. Jain and K. Deb, “An evolutionary many-objective optimization algorithm using reference-point based non-dominated sorting approach, part II: Handling constraints and extending to an adaptive approach,” IEEE Trans. Evolut. Comput., vol. 18, no. 4, pp. 602–622, 2013. doi: 10.1109/TEVC.2013.2281534. [Google Scholar] [CrossRef]
37. M. G. Parsons and R. L. Scott, “Formulation of multi-criterion design optimization problems for solution with scalar numerical optimization methods,” J. Ship. Res., vol. 48, no. 1, pp. 61–76, 2004. doi: 10.5957/jsr.2004.48.1.61. [Google Scholar] [CrossRef]
38. T. Ray, K. Tai, and K. C. Seow, “Multi-objective design optimization by an evolutionary algorithm,” Eng. Optimiz., vol. 33, no. 4, pp. 399–424, 2001. [Google Scholar]
Supplementary Materials
Cite This Article
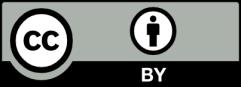