Open Access
ARTICLE
A Health State Prediction Model Based on Belief Rule Base and LSTM for Complex Systems
High-Tech Institute of Xi’an, Xi’an, 710025, China
* Corresponding Author: Zhijie Zhou. Email:
(This article belongs to the Special Issue: Intelligent reasoning and decision-making towards the explainability of AI)
Intelligent Automation & Soft Computing 2024, 39(1), 73-91. https://doi.org/10.32604/iasc.2024.042285
Received 25 May 2023; Accepted 11 September 2023; Issue published 29 March 2024
Abstract
In industrial production and engineering operations, the health state of complex systems is critical, and predicting it can ensure normal operation. Complex systems have many monitoring indicators, complex coupling structures, non-linear and time-varying characteristics, so it is a challenge to establish a reliable prediction model. The belief rule base (BRB) can fuse observed data and expert knowledge to establish a nonlinear relationship between input and output and has well modeling capabilities. Since each indicator of the complex system can reflect the health state to some extent, the BRB is built based on the causal relationship between system indicators and the health state to achieve the prediction. A health state prediction model based on BRB and long short term memory for complex systems is proposed in this paper. Firstly, the LSTM is introduced to predict the trend of the indicators in the system. Secondly, the Density Peak Clustering (DPC) algorithm is used to determine referential values of indicators for BRB, which effectively offset the lack of expert knowledge. Then, the predicted values and expert knowledge are fused to construct BRB to predict the health state of the systems by inference. Finally, the effectiveness of the model is verified by a case study of a certain vehicle hydraulic pump.Keywords
Cite This Article
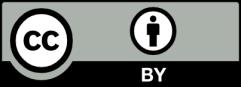