Open Access
ARTICLE
Deep Learning Model for News Quality Evaluation Based on Explicit and Implicit Information
1 State Key Laboratory of Media Convergence and Communication, Communication University of China, Beijing, 100024, China
2 School of Computer and Cyber Sciences, Communication University of China, Beijing, 100024, China
* Corresponding Author: Yongbin Wang. Email:
(This article belongs to the Special Issue: Applying Computational Intelligence to Social Science Research)
Intelligent Automation & Soft Computing 2023, 38(3), 275-295. https://doi.org/10.32604/iasc.2023.041873
Received 09 May 2023; Accepted 19 July 2023; Issue published 27 February 2024
Abstract
Recommending high-quality news to users is vital in improving user stickiness and news platforms’ reputation. However, existing news quality evaluation methods, such as clickbait detection and popularity prediction, are challenging to reflect news quality comprehensively and concisely. This paper defines news quality as the ability of news articles to elicit clicks and comments from users, which represents whether the news article can attract widespread attention and discussion. Based on the above definition, this paper first presents a straightforward method to measure news quality based on the comments and clicks of news and defines four news quality indicators. Then, the dataset can be labeled automatically by the method. Next, this paper proposes a deep learning model that integrates explicit and implicit news information for news quality evaluation (EINQ). The explicit information includes the headline, source, and publishing time of the news, which attracts users to click. The implicit information refers to the news article’s content which attracts users to comment. The implicit and explicit information affect users’ click and comment behavior differently. For modeling explicit information, the typical convolution neural network (CNN) is used to get news headline semantic representation. For modeling implicit information, a hierarchical attention network (HAN) is exploited to extract news content semantic representation while using the latent Dirichlet allocation (LDA) model to get the subject distribution of news as a semantic supplement. Considering the different roles of explicit and implicit information for quality evaluation, the EINQ exploits an attention layer to fuse them dynamically. The proposed model yields the Accuracy of 82.31% and the F-Score of 80.51% on the real-world dataset from Toutiao, which shows the effectiveness of explicit and implicit information dynamic fusion and demonstrates performance improvements over a variety of baseline models in news quality evaluation. This work provides empirical evidence for explicit and implicit factors in news quality evaluation and a new idea for news quality evaluation.Keywords
Cite This Article
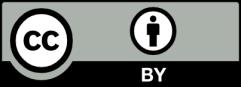
This work is licensed under a Creative Commons Attribution 4.0 International License , which permits unrestricted use, distribution, and reproduction in any medium, provided the original work is properly cited.