Open Access
ARTICLE
Person Re-Identification with Model-Contrastive Federated Learning in Edge-Cloud Environment
1 School of Computer and Software, Nanjing University of Information Science and Technology, Nanjing, 210044, China
2 Jiangsu Collaborative Innovation Center of Atmospheric Environment and Equipment Technology (CICAEET), Nanjing University of Information Science and Technology, Nanjing, 210044, China
3 School of Big Data and Intelligence Engineering, Southwest Forestry University, Kunming, 650233, China
4 Nanjing Meteorological Service Center, Nanjing Meteorological Bureau, Nanjing, 210019, China
* Corresponding Author: Song Wang. Email:
# Baixuan Tang and Xiaolong Xu are co-first authors to this work
Intelligent Automation & Soft Computing 2023, 38(1), 35-55. https://doi.org/10.32604/iasc.2023.036715
Received 10 October 2022; Accepted 06 January 2023; Issue published 26 January 2024
Abstract
Person re-identification (ReID) aims to recognize the same person in multiple images from different camera views. Training person ReID models are time-consuming and resource-intensive; thus, cloud computing is an appropriate model training solution. However, the required massive personal data for training contain private information with a significant risk of data leakage in cloud environments, leading to significant communication overheads. This paper proposes a federated person ReID method with model-contrastive learning (MOON) in an edge-cloud environment, named FRM. Specifically, based on federated partial averaging, MOON warmup is added to correct the local training of individual edge servers and improve the model’s effectiveness by calculating and back-propagating a model-contrastive loss, which represents the similarity between local and global models. In addition, we propose a lightweight person ReID network, named multi-branch combined depth space network (MB-CDNet), to reduce the computing resource usage of the edge device when training and testing the person ReID model. MB-CDNet is a multi-branch version of combined depth space network (CDNet). We add a part branch and a global branch on the basis of CDNet and introduce an attention pyramid to improve the performance of the model. The experimental results on open-access person ReID datasets demonstrate that FRM achieves better performance than existing baseline.Keywords
Cite This Article
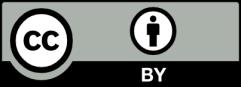
This work is licensed under a Creative Commons Attribution 4.0 International License , which permits unrestricted use, distribution, and reproduction in any medium, provided the original work is properly cited.