Open Access
ARTICLE
Contamination Identification of Lentinula Edodes Logs Based on Improved YOLOv5s
1 School of Information Science & Engineering, Shandong Agricultural University, Tai’an, 271018, China
2 Department of Regulations, Tai’an Service Center for Urban Comprehensive Management, Tai’an, 271018, China
* Corresponding Author: Qiulan Wu. Email:
Intelligent Automation & Soft Computing 2023, 37(3), 3143-3157. https://doi.org/10.32604/iasc.2023.040903
Received 03 April 2023; Accepted 02 June 2023; Issue published 11 September 2023
Abstract
In order to improve the accuracy and efficiency of Lentinula edodes logs contamination identification, an improved YOLOv5s contamination identification model for Lentinula edodes logs (YOLOv5s-CGGS) is proposed in this paper. Firstly, a CA (coordinate attention) mechanism is introduced in the feature extraction network of YOLOv5s to improve the identifiability of Lentinula edodes logs contamination and the accuracy of target localization. Then, the CIoU (Complete-IOU) loss function is replaced by an SIoU (SCYLLA-IoU) loss function to improve the model’s convergence speed and inference accuracy. Finally, the GSConv and GhostConv modules are used to improve and optimize the feature fusion network to improve identification efficiency. The method in this paper achieves values of 97.83%, 97.20%, and 98.20% in precision, recall, and mAP@0.5, which are 2.33%, 3.0%, and 1.5% better than YOLOv5s, respectively. mAP@0.5 is better than YOLOv4, Ghost-YOLOv4, and Mobilenetv3-YOLOv4 (improved by 4.61%, 5.16%, and 6.04%, respectively), and the FPS increased by two to three times.Keywords
Cite This Article
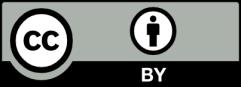
This work is licensed under a Creative Commons Attribution 4.0 International License , which permits unrestricted use, distribution, and reproduction in any medium, provided the original work is properly cited.