Open Access
ARTICLE
A Novel Attack on Complex APUFs Using the Evolutionary Deep Convolutional Neural Network
1 Department of Industrial Engineering, Sharif University of Technology, Tehran, 14588-89694, Iran
2 Department of Business Administration, Boston University, Boston, MA, 02215, USA
3 Department of Industrial Engineering, University of Houston, Houston, TX, 77204, USA
4 ETSI de Telecomunicación, Universidad Politécnica de Madrid, Madrid, 28040, Spain
* Corresponding Author: Diego Martín. Email:
Intelligent Automation & Soft Computing 2023, 37(3), 3059-3081. https://doi.org/10.32604/iasc.2023.040502
Received 21 March 2023; Accepted 12 June 2023; Issue published 11 September 2023
Abstract
As the internet of things (IoT) continues to expand rapidly, the significance of its security concerns has grown in recent years. To address these concerns, physical unclonable functions (PUFs) have emerged as valuable tools for enhancing IoT security. PUFs leverage the inherent randomness found in the embedded hardware of IoT devices. However, it has been shown that some PUFs can be modeled by attackers using machine-learning-based approaches. In this paper, a new deep learning (DL)-based modeling attack is introduced to break the resistance of complex XAPUFs. Because training DL models is a problem that falls under the category of NP-hard problems, there has been a significant increase in the use of meta-heuristics (MH) to optimize DL parameters. Nevertheless, it is widely recognized that finding the right balance between exploration and exploitation when dealing with complex problems can pose a significant challenge. To address these challenges, a novel migration-based multi-parent genetic algorithm (MBMPGA) is developed to train the deep convolutional neural network (DCNN) in order to achieve a higher rate of accuracy and convergence speed while decreasing the run-time of the attack. In the proposed MBMPGA, a non-linear migration model of the biogeography-based optimization (BBO) is utilized to enhance the exploitation ability of GA. A new multi-parent crossover is then introduced to enhance the exploration ability of GA. The behavior of the proposed MBMPGA is examined on two real-world optimization problems. In benchmark problems, MBMPGA outperforms other MH algorithms in convergence rate. The proposed model are also compared with previous attacking models on several simulated challenge-response pairs (CRPs). The simulation results on the XAPUF datasets show that the introduced attack in this paper obtains more than 99% modeling accuracy even on 8-XAPUF. In addition, the proposed MBMPGA-DCNN outperforms the state-of-the-art modeling attacks in a reduced timeframe and with a smaller number of required sets of CRPs. The area under the curve (AUC) of MBMPGA-DCNN outperforms other architectures. MBMPGA-DCNN achieved sensitivities, specificities, and accuracies of 99.12%, 95.14%, and 98.21%, respectively, in the test datasets, establishing it as the most successful method.Keywords
Cite This Article
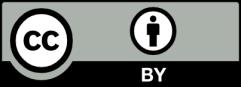
This work is licensed under a Creative Commons Attribution 4.0 International License , which permits unrestricted use, distribution, and reproduction in any medium, provided the original work is properly cited.