Open Access
ARTICLE
Distributed Active Partial Label Learning
1 Key Laboratory of Intelligent Informatics for Safety & Emergency of Zhejiang Province, Wenzhou University, Wenzhou, 325006, China
2 College of Computer Science and Artificial Intelligence Engineering, Wenzhou University, Wenzhou, 325006, China
* Corresponding Author: Weibin Chen. Email:
Intelligent Automation & Soft Computing 2023, 37(3), 2627-2650. https://doi.org/10.32604/iasc.2023.040497
Received 20 March 2023; Accepted 29 June 2023; Issue published 11 September 2023
Abstract
Active learning (AL) trains a high-precision predictor model from small numbers of labeled data by iteratively annotating the most valuable data sample from an unlabeled data pool with a class label throughout the learning process. However, most current AL methods start with the premise that the labels queried at AL rounds must be free of ambiguity, which may be unrealistic in some real-world applications where only a set of candidate labels can be obtained for selected data. Besides, most of the existing AL algorithms only consider the case of centralized processing, which necessitates gathering together all the unlabeled data in one fusion center for selection. Considering that data are collected/stored at different nodes over a network in many real-world scenarios, distributed processing is chosen here. In this paper, the issue of distributed classification of partially labeled (PL) data obtained by a fully decentralized AL method is focused on, and a distributed active partial label learning (dAPLL) algorithm is proposed. Our proposed algorithm is composed of a fully decentralized sample selection strategy and a distributed partial label learning (PLL) algorithm. During the sample selection process, both the uncertainty and representativeness of the data are measured based on the global cluster centers obtained by a distributed clustering method, and the valuable samples are chosen in turn. Meanwhile, using the disambiguation-free strategy, a series of binary classification problems can be constructed, and the corresponding cost-sensitive classifiers can be cooperatively trained in a distributed manner. The experiment results conducted on several datasets demonstrate that the performance of the dAPLL algorithm is comparable to that of the corresponding centralized method and is superior to the existing active PLL (APLL) method in different parameter configurations. Besides, our proposed algorithm outperforms several current PLL methods using the random selection strategy, especially when only small amounts of data are selected to be assigned with the candidate labels.Keywords
Cite This Article
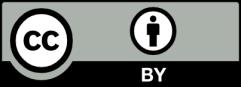
This work is licensed under a Creative Commons Attribution 4.0 International License , which permits unrestricted use, distribution, and reproduction in any medium, provided the original work is properly cited.