Open Access
ARTICLE
Integrated Generative Adversarial Network and XGBoost for Anomaly Processing of Massive Data Flow in Dispatch Automation Systems
1 Nanjing Power Supply Company of State Grid Jiangsu Electric Power Co., Ltd., Nanjing, 210019, China
2 State Grid Jiangsu Electric Power Co., Ltd., Nanjing, 210008, China
* Corresponding Author: Yingqi Liao. Email:
Intelligent Automation & Soft Computing 2023, 37(3), 2825-2848. https://doi.org/10.32604/iasc.2023.039618
Received 08 February 2023; Accepted 06 June 2023; Issue published 11 September 2023
Abstract
Existing power anomaly detection is mainly based on a pattern matching algorithm. However, this method requires a lot of manual work, is time-consuming, and cannot detect unknown anomalies. Moreover, a large amount of labeled anomaly data is required in machine learning-based anomaly detection. Therefore, this paper proposes the application of a generative adversarial network (GAN) to massive data stream anomaly identification, diagnosis, and prediction in power dispatching automation systems. Firstly, to address the problem of the small amount of anomaly data, a GAN is used to obtain reliable labeled datasets for fault diagnosis model training based on a few labeled data points. Then, a two-step detection process is designed for the characteristics of grid anomalies, where the generated samples are first input to the XGBoost recognition system to identify the large class of anomalies in the first step. Thereafter, the data processed in the first step are input to the joint model of Convolutional Neural Networks (CNN) and Long short-term memory (LSTM) for fine-grained analysis to detect the small class of anomalies in the second step. Extensive experiments show that our work can reduce a lot of manual work and outperform the state-of-art anomalies classification algorithms for power dispatching data network.Keywords
Cite This Article
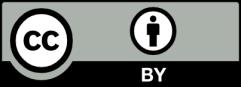
This work is licensed under a Creative Commons Attribution 4.0 International License , which permits unrestricted use, distribution, and reproduction in any medium, provided the original work is properly cited.