Open Access
ARTICLE
Adaptive Multi-Updating Strategy Based Particle Swarm Optimization
1 School of Computer Science and Technology, Kashi University, Kashi, 844006, China
2 Key Laboratory of Intelligent Information Processing, Institute of Computing Technology, Chinese Academy of Sciences, Beijing, 100190, China
* Corresponding Author: Dongping Tian. Email:
Intelligent Automation & Soft Computing 2023, 37(3), 2783-2807. https://doi.org/10.32604/iasc.2023.039531
Received 03 February 2023; Accepted 04 May 2023; Issue published 11 September 2023
Abstract
Particle swarm optimization (PSO) is a stochastic computation technique that has become an increasingly important branch of swarm intelligence optimization. However, like other evolutionary algorithms, PSO also suffers from premature convergence and entrapment into local optima in dealing with complex multimodal problems. Thus this paper puts forward an adaptive multi-updating strategy based particle swarm optimization (abbreviated as AMS-PSO). To start with, the chaotic sequence is employed to generate high-quality initial particles to accelerate the convergence rate of the AMS-PSO. Subsequently, according to the current iteration, different update schemes are used to regulate the particle search process at different evolution stages. To be specific, two different sets of velocity update strategies are utilized to enhance the exploration ability in the early evolution stage while the other two sets of velocity update schemes are applied to improve the exploitation capability in the later evolution stage. Followed by the unequal weightage of acceleration coefficients is used to guide the search for the global worst particle to enhance the swarm diversity. In addition, an auxiliary update strategy is exclusively leveraged to the global best particle for the purpose of ensuring the convergence of the PSO method. Finally, extensive experiments on two sets of well-known benchmark functions bear out that AMS-PSO outperforms several state-of-the-art PSOs in terms of solution accuracy and convergence rate.Keywords
Cite This Article
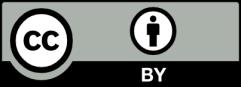