Open Access
ARTICLE
Advanced Guided Whale Optimization Algorithm for Feature Selection in BlazePose Action Recognition
1 Electronic and Electrical Engineering Department, University College London, London, WC1E7JE, England
2 Department of Communications and Electronics, Delta Higher Institute of Engineering and Technology, Mansoura, 35111, Egypt
* Corresponding Author: Motasem S. Alsawadi. Email:
(This article belongs to the Special Issue: Optimization Algorithm for Intelligent Computing Application)
Intelligent Automation & Soft Computing 2023, 37(3), 2767-2782. https://doi.org/10.32604/iasc.2023.039440
Received 30 January 2023; Accepted 24 May 2023; Issue published 11 September 2023
Abstract
The BlazePose, which models human body skeletons as spatiotemporal graphs, has achieved fantastic performance in skeleton-based action identification. Skeleton extraction from photos for mobile devices has been made possible by the BlazePose system. A Spatial-Temporal Graph Convolutional Network (STGCN) can then forecast the actions. The Spatial-Temporal Graph Convolutional Network (STGCN) can be improved by simply replacing the skeleton input data with a different set of joints that provide more information about the activity of interest. On the other hand, existing approaches require the user to manually set the graph’s topology and then fix it across all input layers and samples. This research shows how to use the Statistical Fractal Search (SFS)-Guided whale optimization algorithm (GWOA). To get the best solution for the GWOA, we adopt the SFS diffusion algorithm, which uses the random walk with a Gaussian distribution method common to growing systems. Continuous values are transformed into binary to apply to the feature-selection problem in conjunction with the BlazePose skeletal topology and stochastic fractal search to construct a novel implementation of the BlazePose topology for action recognition. In our experiments, we employed the Kinetics and the NTU-RGB+D datasets. The achieved actiona accuracy in the X-View is 93.14% and in the X-Sub is 96.74%. In addition, the proposed model performs better in numerous statistical tests such as the Analysis of Variance (ANOVA), Wilcoxon signed-rank test, histogram, and times analysis.Keywords
Cite This Article
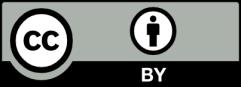
This work is licensed under a Creative Commons Attribution 4.0 International License , which permits unrestricted use, distribution, and reproduction in any medium, provided the original work is properly cited.