Open Access
ARTICLE
A Consistent Mistake in Remote Sensing Images’ Classification Literature
School of Geography Science and Tourism, Hunan University of Arts and Science, Changde, 415000, China
* Corresponding Author: Huaxiang Song. Email:
Intelligent Automation & Soft Computing 2023, 37(2), 1381-1398. https://doi.org/10.32604/iasc.2023.039315
Received 21 January 2023; Accepted 10 March 2023; Issue published 21 June 2023
Abstract
Recently, the convolutional neural network (CNN) has been dominant in studies on interpreting remote sensing images (RSI). However, it appears that training optimization strategies have received less attention in relevant research. To evaluate this problem, the author proposes a novel algorithm named the Fast Training CNN (FST-CNN). To verify the algorithm’s effectiveness, twenty methods, including six classic models and thirty architectures from previous studies, are included in a performance comparison. The overall accuracy (OA) trained by the FST-CNN algorithm on the same model architecture and dataset is treated as an evaluation baseline. Results show that there is a maximal OA gap of 8.35% between the FST-CNN and those methods in the literature, which means a 10% margin in performance. Meanwhile, all those complex roadmaps, e.g., deep feature fusion, model combination, model ensembles, and human feature engineering, are not as effective as expected. It reveals that there was systemic suboptimal performance in the previous studies. Most of the CNN-based methods proposed in the previous studies show a consistent mistake, which has made the model’s accuracy lower than its potential value. The most important reasons seem to be the inappropriate training strategy and the shift in data distribution introduced by data augmentation (DA). As a result, most of the performance evaluation was conducted based on an inaccurate, suboptimal, and unfair result. It has made most of the previous research findings questionable to some extent. However, all these confusing results also exactly demonstrate the effectiveness of FST-CNN. This novel algorithm is model-agnostic and can be employed on any image classification model to potentially boost performance. In addition, the results also show that a standardized training strategy is indeed very meaningful for the research tasks of the RSI-SC.Keywords
Cite This Article
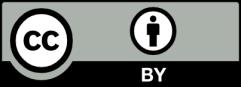