Open Access
ARTICLE
FedNRM: A Federal Personalized News Recommendation Model Achieving User Privacy Protection
1 School of Computer Science and Technology, Donghua University, Shanghai, 201620, China
2 School of Information Engineering, Huzhou University, Huzhou, 313000, China
* Corresponding Author: Shigen Shen. Email:
Intelligent Automation & Soft Computing 2023, 37(2), 1729-1751. https://doi.org/10.32604/iasc.2023.039911
Received 24 February 2023; Accepted 17 April 2023; Issue published 21 June 2023
Abstract
In recent years, the type and quantity of news are growing rapidly, and it is not easy for users to find the news they are interested in the massive amount of news. A news recommendation system can score and predict the candidate news, and finally recommend the news with high scores to users. However, existing user models usually only consider users’ long-term interests and ignore users’ recent interests, which affects users’ usage experience. Therefore, this paper introduces gated recurrent unit (GRU) sequence network to capture users’ short-term interests and combines users’ short-term interests and long-term interests to characterize users. While existing models often only use the user’s browsing history and ignore the variability of different users’ interest in the same news, we introduce additional user’s ID information and apply the personalized attention mechanism for user representation. Thus, we achieve a more accurate user representation. We also consider the risk of compromising user privacy if the user model training is placed on the server side. To solve this problem, we design the training of the user model locally on the client side by introducing a federated learning framework to keep the user’s browsing history on the client side. We further employ secure multi-party computation to request news representations from the server side, which protects privacy to some extent. Extensive experiments on a real-world news dataset show that our proposed news recommendation model has a better improvement in several performance evaluation metrics. Compared with the current state-of-the-art federated news recommendation models, our model has increased by 0.54% in AUC, 1.97% in MRR, 2.59% in nDCG@5%, and 1.89% in nDCG@10. At the same time, because we use a federated learning framework, compared with other centralized news recommendation methods, we achieve privacy protection for users.Keywords
Cite This Article
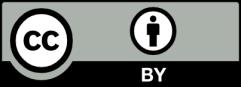
This work is licensed under a Creative Commons Attribution 4.0 International License , which permits unrestricted use, distribution, and reproduction in any medium, provided the original work is properly cited.