Open Access
ARTICLE
Predicting Lumbar Spondylolisthesis: A Hybrid Deep Learning Approach
1 Department of Computer Application, SAGE University, Indore, 452012, Madhya Pradesh, India
2 IAC, SAGE University, Indore, 452012, Madhya Pradesh, India
3 Shivang College of Physiotherapy, Ratlam, 4570021, Madhya Pradesh, India
4 Medi-Caps University, Rau Indore, Madhya Pradesh, 453331, India
5 Chitkara University Institute of Engineering and Technology, Chitkara University, Punjab, India
6 Department of Electronics and Communication Engineering, Kuwait College of Science and Technology (KCST), Doha, Kuwait
7 Department of Computer Science and Engineering, Sreenivasa Institute of Technology and Management Studies, Chittoor, Andra Pradesh, India
* Corresponding Authors: Shweta Agrawal. Email: ; Abolfazl Mehbodniya. Email:
(This article belongs to the Special Issue: Intelligent Systems for Diversified Application Domains)
Intelligent Automation & Soft Computing 2023, 37(2), 2133-2151. https://doi.org/10.32604/iasc.2023.039836
Received 20 February 2023; Accepted 14 April 2023; Issue published 21 June 2023
Abstract
Spondylolisthesis is a chronic disease, and a timely diagnosis of it may help in avoiding surgery. Disease identification in x-ray radiographs is very challenging. Strengthening the feature extraction tool in VGG16 has improved the classification rate. But the fully connected layers of VGG16 are not efficient at capturing the positional structure of an object in images. Capsule network (CapsNet) works with capsules (neuron clusters) rather than a single neuron to grasp the properties of the provided image to match the pattern. In this study, an integrated model that is a combination of VGG16 and CapsNet (S-VCNet) is proposed. In the model, VGG16 is used as a feature extractor. After feature extraction, the output is fed to CapsNet for disease identification. A private dataset is used that contains 466 X-ray radiographs, including 186 images displaying a spine with spondylolisthesis and 280 images depicting a normal spine. The suggested model is the first step towards developing a web-based radiological diagnosis tool that can be utilized in outpatient clinics where there are not enough qualified medical professionals. Experimental results demonstrate that the developed model outperformed the other models that are used for lumbar spondylolisthesis diagnosis with 98% accuracy. After the performance check, the model has been successfully deployed on the Gradio web app platform to produce the outcome in less than 20 s.Keywords
Cite This Article
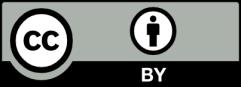
This work is licensed under a Creative Commons Attribution 4.0 International License , which permits unrestricted use, distribution, and reproduction in any medium, provided the original work is properly cited.