Open Access
ARTICLE
Ensemble-Based Approach for Efficient Intrusion Detection in Network Traffic
1 School of Computing, Skyline University College, University City of Sharjah, P. O. Box 1797, Sharjah, United Arab Emirates
2 IT-Department-Al-Huson University College, Al-Balqa Applied University, P. O. Box 50, Irbid, Jordan
3 Information Systems Department, College of Computing & Informatics, University of Sharjah, United Arab Emirates
4 Comp. Info Sciences (CIS) Division, Higher Colleges of Technology, Sharjah, United Arab Emirates
5 Computer Sciences Department, Yarmouk University, Irbid, Jordan
6 Computer Network and Information Systems Department, The World Islamic Sciences and Education University, Amman, 11947, Jordan
7 Department of Information Security, Faculty of Information Technology, University of Petra, Amman, Jordan
* Corresponding Author: Ammar Almomani. Email:
Intelligent Automation & Soft Computing 2023, 37(2), 2499-2517. https://doi.org/10.32604/iasc.2023.039687
Received 11 February 2023; Accepted 18 May 2023; Issue published 21 June 2023
Abstract
The exponential growth of Internet and network usage has necessitated heightened security measures to protect against data and network breaches. Intrusions, executed through network packets, pose a significant challenge for firewalls to detect and prevent due to the similarity between legitimate and intrusion traffic. The vast network traffic volume also complicates most network monitoring systems and algorithms. Several intrusion detection methods have been proposed, with machine learning techniques regarded as promising for dealing with these incidents. This study presents an Intrusion Detection System Based on Stacking Ensemble Learning base (Random Forest, Decision Tree, and k-Nearest-Neighbors). The proposed system employs pre-processing techniques to enhance classification efficiency and integrates seven machine learning algorithms. The stacking ensemble technique increases performance by incorporating three base models (Random Forest, Decision Tree, and k-Nearest-Neighbors) and a meta-model represented by the Logistic Regression algorithm. Evaluated using the UNSW-NB15 dataset, the proposed IDS gained an accuracy of 96.16% in the training phase and 97.95% in the testing phase, with precision of 97.78%, and 98.40% for taring and testing, respectively. The obtained results demonstrate improvements in other measurement criteria.Keywords
Cite This Article
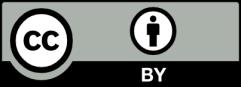
This work is licensed under a Creative Commons Attribution 4.0 International License , which permits unrestricted use, distribution, and reproduction in any medium, provided the original work is properly cited.