Open Access
ARTICLE
Two-Layer Information Granulation: Mapping-Equivalence Neighborhood Rough Set and Its Attribute Reduction
1 School of Computer, Jiangsu University of Science and Technology, Zhenjiang, 212100, China
2 Key Laboratory of Oceanographic Big Data Mining & Application of Zhejiang Province, Zhejiang Ocean University, Zhoushan, 316022, China
* Corresponding Author: Jingjing Song. Email:
(This article belongs to the Special Issue: Cognitive Granular Computing Methods for Big Data Analysis)
Intelligent Automation & Soft Computing 2023, 37(2), 2059-2075. https://doi.org/10.32604/iasc.2023.039592
Received 07 February 2023; Accepted 23 April 2023; Issue published 21 June 2023
Abstract
Attribute reduction, as one of the essential applications of the rough set, has attracted extensive attention from scholars. Information granulation is a key step of attribute reduction, and its efficiency has a significant impact on the overall efficiency of attribute reduction. The information granulation of the existing neighborhood rough set models is usually a single layer, and the construction of each information granule needs to search all the samples in the universe, which is inefficient. To fill such gap, a new neighborhood rough set model is proposed, which aims to improve the efficiency of attribute reduction by means of two-layer information granulation. The first layer of information granulation constructs a mapping-equivalence relation that divides the universe into multiple mutually independent mapping-equivalence classes. The second layer of information granulation views each mapping-equivalence class as a sub-universe and then performs neighborhood information granulation. A model named mapping-equivalence neighborhood rough set model is derived from the strategy of two-layer information granulation. Experimental results show that compared with other neighborhood rough set models, this model can effectively improve the efficiency of attribute reduction and reduce the uncertainty of the system. The strategy provides a new thinking for the exploration of neighborhood rough set models and the study of attribute reduction acceleration problems.Keywords
Cite This Article
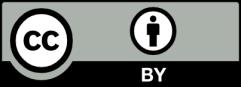
This work is licensed under a Creative Commons Attribution 4.0 International License , which permits unrestricted use, distribution, and reproduction in any medium, provided the original work is properly cited.