Open Access
ARTICLE
An Update Method of Decision Implication Canonical Basis on Attribute Granulating
1 School of Computer and Information Technology, Shanxi University, Taiyuan, Shanxi Province, 030006, China
2 Key Laboratory of Computational Intelligence and Chinese Information Processing of Ministry of Education, Shanxi University, Taiyuan, Shanxi Province, 030006, China
* Corresponding Author: Yanhui Zhai. Email:
(This article belongs to the Special Issue: Cognitive Granular Computing Methods for Big Data Analysis)
Intelligent Automation & Soft Computing 2023, 37(2), 1833-1851. https://doi.org/10.32604/iasc.2023.039553
Received 04 February 2023; Accepted 11 April 2023; Issue published 21 June 2023
Abstract
Decision implication is a form of decision knowledge representation, which is able to avoid generating attribute implications that occur between condition attributes and between decision attributes. Compared with other forms of decision knowledge representation, decision implication has a stronger knowledge representation capability. Attribute granularization may facilitate the knowledge extraction of different attribute granularity layers and thus is of application significance. Decision implication canonical basis (DICB) is the most compact set of decision implications, which can efficiently represent all knowledge in the decision context. In order to mine all decision information on decision context under attribute granulating, this paper proposes an updated method of DICB. To this end, the paper reduces the update of DICB to the updates of decision premises after deleting an attribute and after adding granulation attributes of some attributes. Based on this, the paper analyzes the changes of decision premises, examines the properties of decision premises, designs an algorithm for incrementally generating DICB, and verifies its effectiveness through experiments. In real life, by using the updated algorithm of DICB, users may obtain all decision knowledge on decision context after attribute granularization.Keywords
Cite This Article
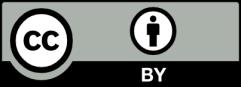
This work is licensed under a Creative Commons Attribution 4.0 International License , which permits unrestricted use, distribution, and reproduction in any medium, provided the original work is properly cited.