Open Access
ARTICLE
Missing Value Imputation Model Based on Adversarial Autoencoder Using Spatiotemporal Feature Extraction
1 Department of Computer Science, Kyonggi University, Suwon-si, Gyeonggi-do, 16227, Korea
2 Department of Public Safety Bigdata, Kyonggi University, Suwon-si, Gyeonggi-do, 16227, Korea
3 Division of AI Computer Science and Engineering, Kyonggi University, Suwon-si, Gyeonggi-do, 16227, Korea
* Corresponding Author: Kyungyong Chung. Email:
Intelligent Automation & Soft Computing 2023, 37(2), 1925-1940. https://doi.org/10.32604/iasc.2023.039317
Received 22 January 2023; Accepted 17 April 2023; Issue published 21 June 2023
Abstract
Recently, the importance of data analysis has increased significantly due to the rapid data increase. In particular, vehicle communication data, considered a significant challenge in Intelligent Transportation Systems (ITS), has spatiotemporal characteristics and many missing values. High missing values in data lead to the decreased predictive performance of models. Existing missing value imputation models ignore the topology of transportation networks due to the structural connection of road networks, although physical distances are close in spatiotemporal image data. Additionally, the learning process of missing value imputation models requires complete data, but there are limitations in securing complete vehicle communication data. This study proposes a missing value imputation model based on adversarial autoencoder using spatiotemporal feature extraction to address these issues. The proposed method replaces missing values by reflecting spatiotemporal characteristics of transportation data using temporal convolution and spatial convolution. Experimental results show that the proposed model has the lowest error rate of 5.92%, demonstrating excellent predictive accuracy. Through this, it is possible to solve the data sparsity problem and improve traffic safety by showing superior predictive performance.Keywords
Cite This Article
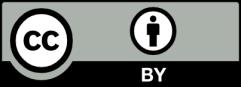
This work is licensed under a Creative Commons Attribution 4.0 International License , which permits unrestricted use, distribution, and reproduction in any medium, provided the original work is properly cited.