Open Access
ARTICLE
A Novel Ensemble Learning System for Cyberattack Classification
Department of Computer and Telematic Systems Engineering, Universidad de Extremadura, School of Technology, Cáceres, 10005, Spain
* Corresponding Author: Óscar Mogollón-Gutiérrez. Email:
Intelligent Automation & Soft Computing 2023, 37(2), 1691-1709. https://doi.org/10.32604/iasc.2023.039255
Received 18 January 2023; Accepted 13 April 2023; Issue published 21 June 2023
Abstract
Nowadays, IT systems rely mainly on artificial intelligence (AI) algorithms to process data. AI is generally used to extract knowledge from stored information and, depending on the nature of data, it may be necessary to apply different AI algorithms. In this article, a novel perspective on the use of AI to ensure the cybersecurity through the study of network traffic is presented. This is done through the construction of a two-stage cyberattack classification ensemble model addressing class imbalance following a one-vs-rest (OvR) approach. With the growing trend of cyberattacks, it is essential to implement techniques that ensure legitimate access to information. To address this issue, this work proposes a network traffic classification system for different categories based on several AI techniques. In the first task, binary models are generated to clearly differentiate each type of traffic from the rest. With binary models generated, an ensemble model is developed in two phases, which allows the separation of legitimate and illegitimate traffic (phase 1) while also identifying the type of illegitimate traffic (phase 2). In this way, the proposed system allows a complete multiclass classification of network traffic. The estimation of global performance is done using a modern dataset (UNSW-NB15), evaluated using two approaches and compared with other state-of-art works. Our proposal, based on the construction of a two-step model, reaches an F1 of 0.912 for the first level of binary classification and 0.7754 for the multiclass classification. These results show that the proposed system outperforms other state-of-the-art approaches (+0.75% and +3.54% for binary and multiclass classification, respectively) in terms of F1, as demonstrated through comparison together with other relevant classification metrics.Keywords
Cite This Article
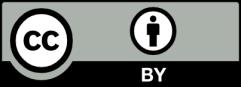
This work is licensed under a Creative Commons Attribution 4.0 International License , which permits unrestricted use, distribution, and reproduction in any medium, provided the original work is properly cited.