Open Access
ARTICLE
Stock Market Index Prediction Using Machine Learning and Deep Learning Techniques
1 Yangtze Delta Region Institute (Huzhou), University of Electronic Science and Technology of China, Huzhou, 313001, China
2 Abdul Wali Khan University Mardan, Mardan, 23200, Pakistan
3 School of Computer Science and Engineering, University of Electronic Science and Technology of China, Chengdu, 611731, China
4 Brain Institute Peshawar, Peshawar, 25130, Pakistan
* Corresponding Authors: Amin ul Haq. Email: ; Rajesh Kumar. Email:
(This article belongs to the Special Issue: Smart digital education and scientific programming)
Intelligent Automation & Soft Computing 2023, 37(2), 1325-1344. https://doi.org/10.32604/iasc.2023.038849
Received 31 December 2022; Accepted 24 February 2023; Issue published 21 June 2023
A correction of this article was approved in:
Correction: Stock Market Index Prediction Using Machine Learning and Deep Learning Techniques
Read correction
Abstract
Stock market forecasting has drawn interest from both economists and computer scientists as a classic yet difficult topic. With the objective of constructing an effective prediction model, both linear and machine learning tools have been investigated for the past couple of decades. In recent years, recurrent neural networks (RNNs) have been observed to perform well on tasks involving sequence-based data in many research domains. With this motivation, we investigated the performance of long-short term memory (LSTM) and gated recurrent units (GRU) and their combination with the attention mechanism; LSTM + Attention, GRU + Attention, and LSTM + GRU + Attention. The methods were evaluated with stock data from three different stock indices: the KSE 100 index, the DSE 30 index, and the BSE Sensex. The results were compared to other machine learning models such as support vector regression, random forest, and k-nearest neighbor. The best results for the three datasets were obtained by the RNN-based models combined with the attention mechanism. The performances of the RNN and attention-based models are higher and would be more effective for applications in the business industry.Keywords
Cite This Article
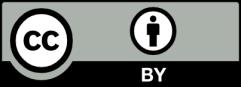
This work is licensed under a Creative Commons Attribution 4.0 International License , which permits unrestricted use, distribution, and reproduction in any medium, provided the original work is properly cited.