Open Access
ARTICLE
Forecasting Energy Consumption Using a Novel Hybrid Dipper Throated Optimization and Stochastic Fractal Search Algorithm
1 Department of Computer Sciences, College of Computer and Information Sciences, Princess Nourah bint Abdulrahman University, P. O. Box 84428, Riyadh, 11671, Saudi Arabia
2 Department of Communications and Electronics, Delta Higher Institute of Engineering and Technology, Mansoura, 35111, Egypt
3 Faculty of Artificial Intelligence, Delta University for Science and Technology, Mansoura, Egypt
* Corresponding Author: Amel Ali Alhussan. Email:
(This article belongs to the Special Issue: Optimization Algorithm for Intelligent Computing Application)
Intelligent Automation & Soft Computing 2023, 37(2), 2117-2132. https://doi.org/10.32604/iasc.2023.038811
Received 30 December 2022; Accepted 10 April 2023; Issue published 21 June 2023
Abstract
The accurate prediction of energy consumption has effective role in decision making and risk management for individuals and governments. Meanwhile, the accurate prediction can be realized using the recent advances in machine learning and predictive models. This research proposes a novel approach for energy consumption forecasting based on a new optimization algorithm and a new forecasting model consisting of a set of long short-term memory (LSTM) units. The proposed optimization algorithm is used to optimize the parameters of the LSTM-based model to boost its forecasting accuracy. This optimization algorithm is based on the recently emerged dipper-throated optimization (DTO) and stochastic fractal search (SFS) algorithm and is referred to as dynamic DTOSFS. To prove the effectiveness and superiority of the proposed approach, five standard benchmark algorithms, namely, stochastic fractal search (SFS), dipper throated optimization (DTO), whale optimization algorithm (WOA), particle swarm optimization (PSO), and grey wolf optimization (GWO), are used to optimize the parameters of the LSTM-based model, and the results are compared with that of the proposed approach. Experimental results show that the proposed DDTOSFS + LSTM can accurately forecast the energy consumption with root mean square error RMSE of 0.00013, which is the best among the recorded results of the other methods. In addition, statistical experiments are conducted to prove the statistical difference of the proposed model. The results of these tests confirmed the expected outcomes.Keywords
Cite This Article
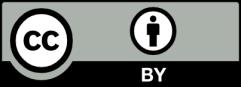
This work is licensed under a Creative Commons Attribution 4.0 International License , which permits unrestricted use, distribution, and reproduction in any medium, provided the original work is properly cited.